AI Compliance at Scale via Embedded Data Governance
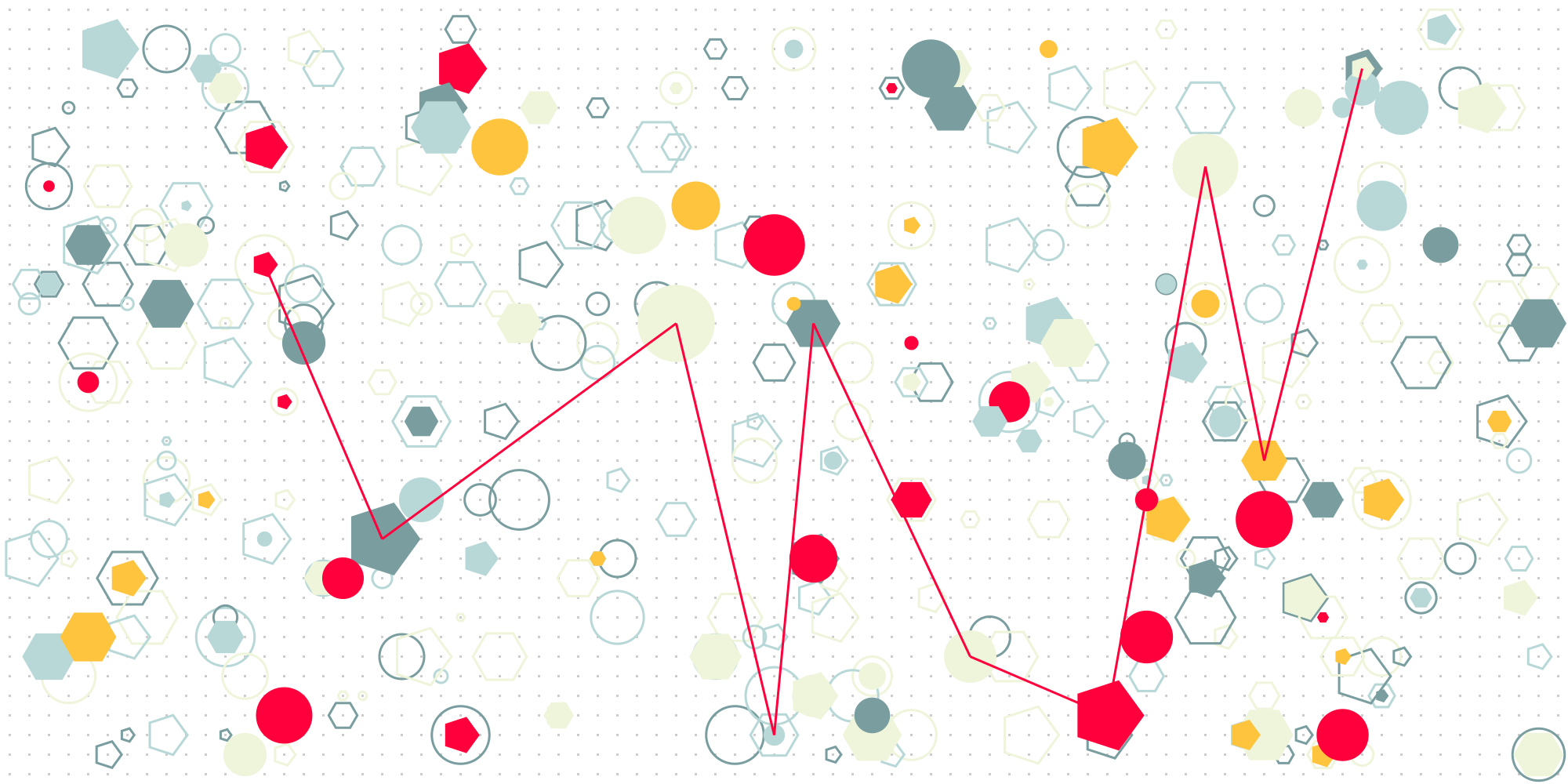
There are, roughly speaking, three problems to solve for an Artificial Intelligence system to comply with AI regulations in China (see the note here) and likely future regulation in the USA (see the notes on the Algorithmic Accountability Act, starting here):
- Data governance of input and output data;
- Explainability, itself involving (i) the ability to trace the output to the subset of input data used to produce it, (ii) documentation of the model trained from the data; and
- Assessment of risks of what outputs the system produces, and how these could be used and misused.
Using available, large-scale crawled web/Internet data is a low-cost (it’s all relative) approach to train an AI system. The problem with it, as I wrote separately, here, is that it makes it hard to claim compliance with regulations requiring basics of data governance. For illustration, think about data lineage, and consider how costly it would be to determine the source of data mentioned in a forum post, which mentions that data without referencing a source, whereby the text of the forum post is used as training data.
The inevitable solution to this is that there should be, and in fact is, demand for datasets which come packaged with data governance – metadata, data quality measures, lineage information, and so on. With demand, there’s supply, and various providers of such datasets are only a Google Search away.
The approach, then, to enabling compliance across many AI systems, is that they use data which is demonstrably governed to at least the bare minimum required by regulations in locations where the systems have customers.
In short, there’s a market for well governed training data.
There are at least three major kinds of datasets, based on where data originates from:
- Datasets or streams originating in somehow selected subsets of crawled web data, to which basics of data governance are applied;
- Datasets or streams of data carefully elicited from people, whereby the value of the data depends on the social importance of people who can be considered as its authors, whereby data needs again to meet minimal data governance requirements;
- Datasets or streams of well governed sensor data, where there is not much of a role for people – e.g., weather data.
In short, scale up data governance will likely be done not by governing massive heterogeneous datasets, but by governing smaller ones, and distribute the effort across specialist dataset providers. Depending on intended use of AI, the AI designer can then select and license only data that is both needed, and well governed.
Scaling up here means that there are thick markets for all these types of datasets. This in turn requires that demand and supply incentives are aligned, a topic for another note.
Another problem is how to stimulate the creation of substitutable datasets, i.e., how to stimulate competition in such a market, as that is possibly the only way to increase training data quality.