Business Forecasts as Verifiable Explanations of Expected Value
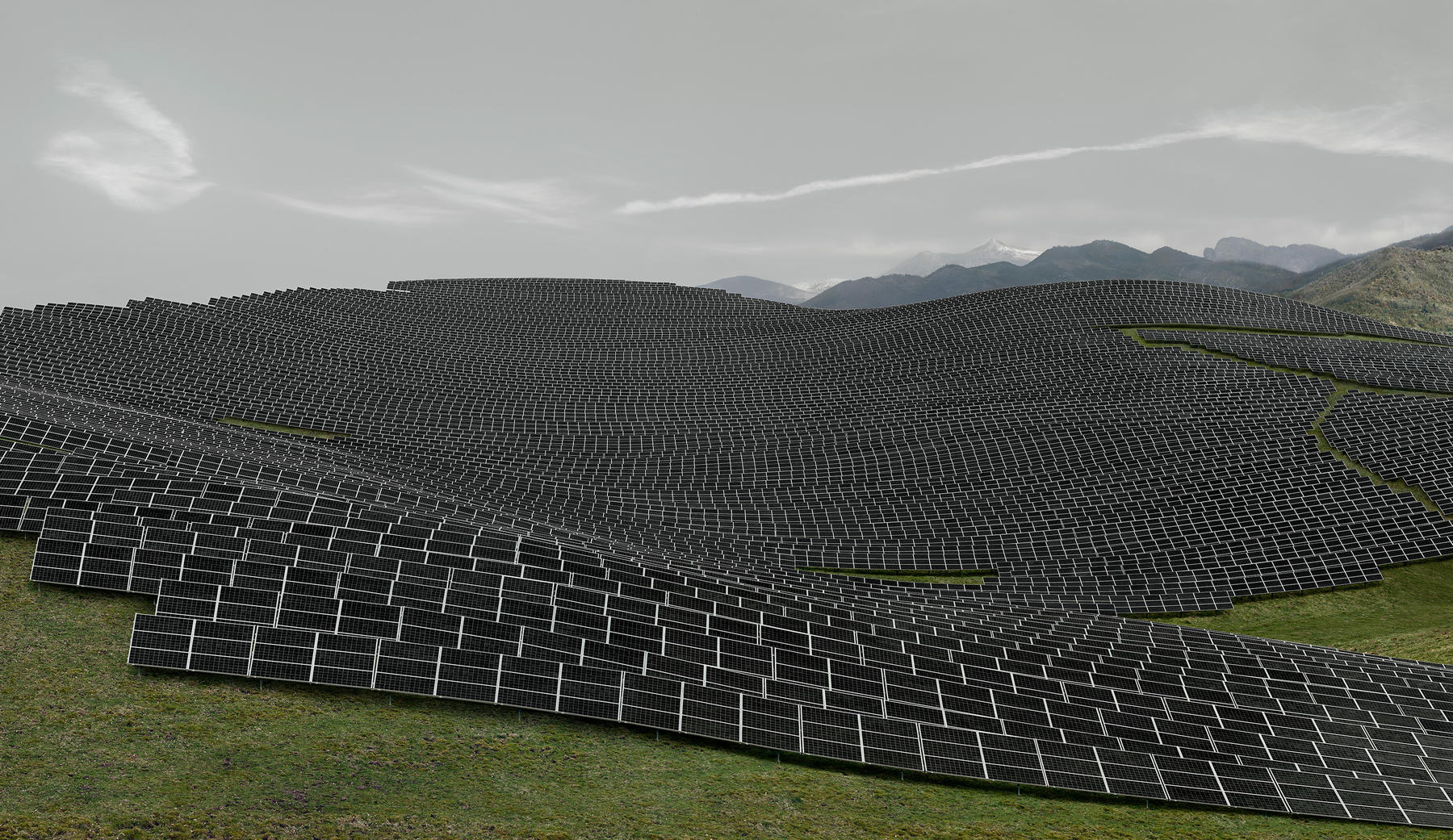
An OECD report [1] estimated that there were about 41,000 publicly traded companies in the world in 2019. Given the usual reporting requirements of listed companies, each maintains a forecast of future conditions that may matter to its financials. In other words, each of these companies has a narrative about a future, according to what its management
- knows and assumes about the mechanisms that influenced past performance,
- if/how these mechanisms will change in the future, and
- what performance they will lead to.
Each narrative is likely to be narrow, focusing on what is believed to influence only the company’s performance: if X trades apples to B2C companies, the forecast will be about the interplay of factors influencing purchasing of apples by these B2C companies; therefore, the forecast is unlikely to be concerned with, say, who will win the next five Champions League seasons (although this may be part of a forecast in a business that’s deciding which of the leading soccer clubs to sponsor).
What if we could combine all these 40+ thousand forecasts into one? Wouldn’t that paint an interesting picture of possible ways that future events could unfold? It would be relevant reading for anyone in each of the companies involved, policy makers, and would feed quality input back to everyone who was involved in forecasting in the first place, helping them iterate and improve their explanations of possible futures.
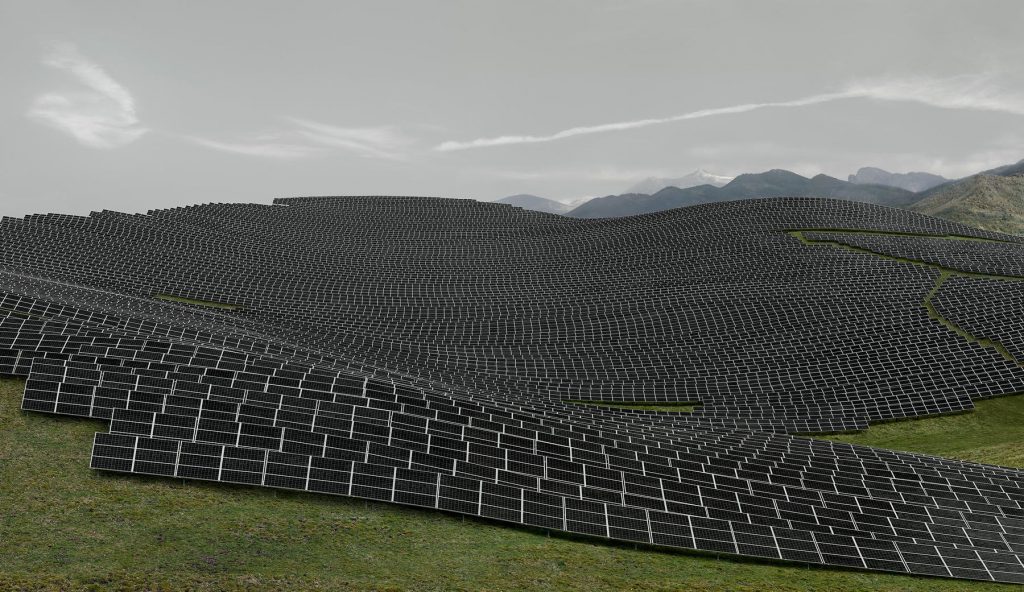
There are two parts to the problem of combining forecasts from many companies:
- Unclear incentives: If company X shares its (redacted) forecast with Y, will that, and how influence actions of Y relative to X (and X’s products, services, staff, and so on). If X and Y are not selling substitutes, this is less of an issue, but even in that case, they may compete for resources, such as competent staff. If X gave a bleak forecast, and Y is optimistic, then this provides an argument to move from X to Y. Forecasts could/would be anonymized, which solves that problem, at least to an extent, since a high degree of anonymity can only be reached by increasingly removing information, thus reducing the quality of redacted/anonymized forecasts being shared.
- Diverse forecast structures: Each company has its own way of forecasting, and each produces a forecast that has structure and content that fits its uses. Having thousands of very different forecasts as input creates another set of difficulties, of translating between them, so that we can identify dependencies as we try to make one integrated forecast out of a multitude of disconnected ones.
To the best of my knowledge, there is no solution at the moment to both parts of the problem. State of the art on practical forecasting is probably (still today) the Superforecasting approach [4,5], where individuals provide forecasts in the form of answers to well-defined questions, can provide free-form comments to justify their choice, and are given feedback after the event occurs, that resolves the question (e.g., when asked “Will team X win championship Y?”, you provide your answer, which makes the forecast, and you learn the actual outcome after the championship ends). Forecasters are rated. In a nutshell, the approach closes the loop of informing the forecaster of the outcome, selects high performers, and qualifies their forecasts as better than the rest.
All in all, Superforecasting solves neither the unclear incentives, nor the diversity of forecast structures. There is no combination of forecasts of different topics, which would be the case when combining forecasts on all sorts of questions (and would be the case if we wanted to combine forecasts from many companies across different sectors). There is also no structure to the forecast in Superforecasting, other than it being about the occurrence of a well-defined event, so that a clear question can be asked. If you look at a random Good Judgment Open challenge, explanations of a forecast are usually absent, and when present, they are unstructured – so while the answers to the forecasting question are summarized, justifications or explanations of answers are not combined. Here is an example, for the question “When will the US Transportation Security Administration (TSA) next screen 2.3 million or more travelers per day for three consecutive days?”
In the rest of this text, I will leave aside the issue of unclear incentives. From the standpoint of economic theory, this is a complicated instance of incentive alignment problems, one that probably could be formulated as an imperfect information game of many actors (as in game theory), with some generalizations of incentives that these actors may have, when taking part in the game of sharing forecasts.
I therefore focus below on the issue with the diverse structures of forecasts. If you think about it, there is no point debating incentives before having an idea of what a forecast would look like, and therefore, what one might want to get or give in return for participating in some incentive mechanism.
This leads to a question of ontology: what is a forecast?
If you are or have been involved in forecasting in business, a forecast is usually a combination of a narrative about whether some events of interest might happen, accompanied by a sequence of positive and negative cash flows that reflect the financial implications of the occurrence of these events. Frequently, there is a bunch of alternative forecasts, i.e., scenarios, and there will be a procedure to select those that a group interested in the forecast likes more than others.
To make this more precise, we can say that a forecast includes at the very least:
- A list of events that are assumed to occur in the future;
- An association of these events to moments or periods of time (i.e., when does each event occur);
- A sequence of cash flows that result from someone’s interpretation of how the occurrence of events relates to estimates of economic value of the outcomes of these events.
The limitation of that approach is that the forecast does not explain the following:
- Why do the selected events matter (rather than some others, which may simply be omitted)?
- Why is it that the occurrence of these events lead to the assumed cash flows?
If we are even more demanding, then the following are missing as well:
- How likely are the events to occur? (Usually, this is quantified by a probability estimate, or a range.)
- Why are they as likely to occur? (Keep in mind that a probability value that describes the uncertainty associated with the occurrence of an event does not substitute for an explanation for why the event may or may not occur, and the confidence that it does either of these.)
As soon as we get into the quantification of uncertainty, more questions come up:
- What assumptions do you make about the processes that generate the occurrence of uncertain events?
- How do you translate these assumptions into properties of the probability distributions that you use to incorporate uncertainty into any calculations of expected value? (Note that expected value here has a technical meaning, an in expected utility theory – in a nutshell, an expected value is value adjusted for the uncertainty of it being realized; if I’m assigning a probability of 0.3 to getting 100 of something, then the expected value is 30.)
A view of all of the above is that we are simply digging a deeper hole by introducing complications – we moved from talking about possible events and the economic implications of their occurrence, to expected value, assumptions about probability distributions, and so on. The nice thing about expected value is that it moves qualitative information about preference and uncertainty into numerical values, and so makes computation easier. Bluntly, it makes it easier to shift from acknowledging the limits of what you know about why business performance is as it is, into calculating how it might turn out to be; a rule of thumb is that the more scenarios someone is giving you, the less they understand what causes, or to be milder, correlates with the events they are forecasting.
A move from qualitative to quantitative can be a mixed blessing, since you really need to know what you are doing, before you take the numbers seriously, i.e., before they make you change your mind when making resource allocation decisions. As Nassim Taleb argued at length in his Incerto books, as did many others before him, it is easy to pick the wrong probability distribution function, or better, it is easy to make wrong assumptions about how an event is uncertain. Put another way, it is easy to pick a probability distribution function to describe the uncertainty in the occurrence of some event, and be fooled into thinking that you understand something about that event precisely because you described uncertainty of its occurrence.
Remember, in business forecasting, one of the basic ideas to keep in mind is that a forecast is perhaps a target, and a target is perhaps a forecast. So a forecast is not a neutral look at possible futures, it mixes assumptions about possible actions, and about how they relate to an environment in which those actions will be taken.
A fairly sophisticated point that is at the core of forecasting, and that is easy to ignore simply because it makes forecasting much, much harder, is that the only time when quantifying uncertainty is acceptable, is after you have an explanation, however limited, of the mechanisms that lead to the occurrence of events that you are interested in.
For example, it is one problem to forecast the values of metrics on a business process that is for the most part under the control of the staff in a company, using equipment belonging to the company, to do something that’s been done many times in the past – i.e., a forecast of a process in which the sources of uncertainty are well understood and can be proactively influenced. It is a very different problem to forecast customer behavior on a new sales channel, for a new set of products. But in either case, the sustainable approach to forecasting (sustainable because it involves incremental construction of knowledge, and one that vaguely resembles scientific method), is to start by trying to explain why events of interest are happening, to build a robust explanation, treat various components of the explanation as hypotheses, and take actions which help validate or invalidate them (ideally, historical data might help validation). Moving from an explanation to probabilities about the occurrence of relevant events is then a simpler step, that can be made with confidence that comes from having a better idea of what we are talking about when we talk about probability.
If this is the approach to forecasting, then everything I wrote about the structure and quality of explanations applies; in this view, a forecast amounts to a verifiable explanation of expected value.
Another major benefit of forecasting based on verifiable explanations is that it makes it easier to integrate these explanations. It amounts to the problem of integrating theories, a topic that requires a separate text.
References
- OECD, 2019, Owners of the World’s Listed Companies.
- Anscombe, Francis J., and Robert J. Aumann. “A definition of subjective probability.” Annals of mathematical statistics 34.1 (1963): 199-205.
- Kahneman, Daniel, and Amos Tversky. “Subjective probability: A judgment of representativeness.” Cognitive psychology 3.3 (1972): 430-454.
- Taleb, Nassim Nicholas. INCERTO: Fooled by Randomness, the Black Swan, the Bed of Procrustes, Antifragile, Skin in the Game. Random House Trade, 2021.