When Is a Career Resilient to AI?
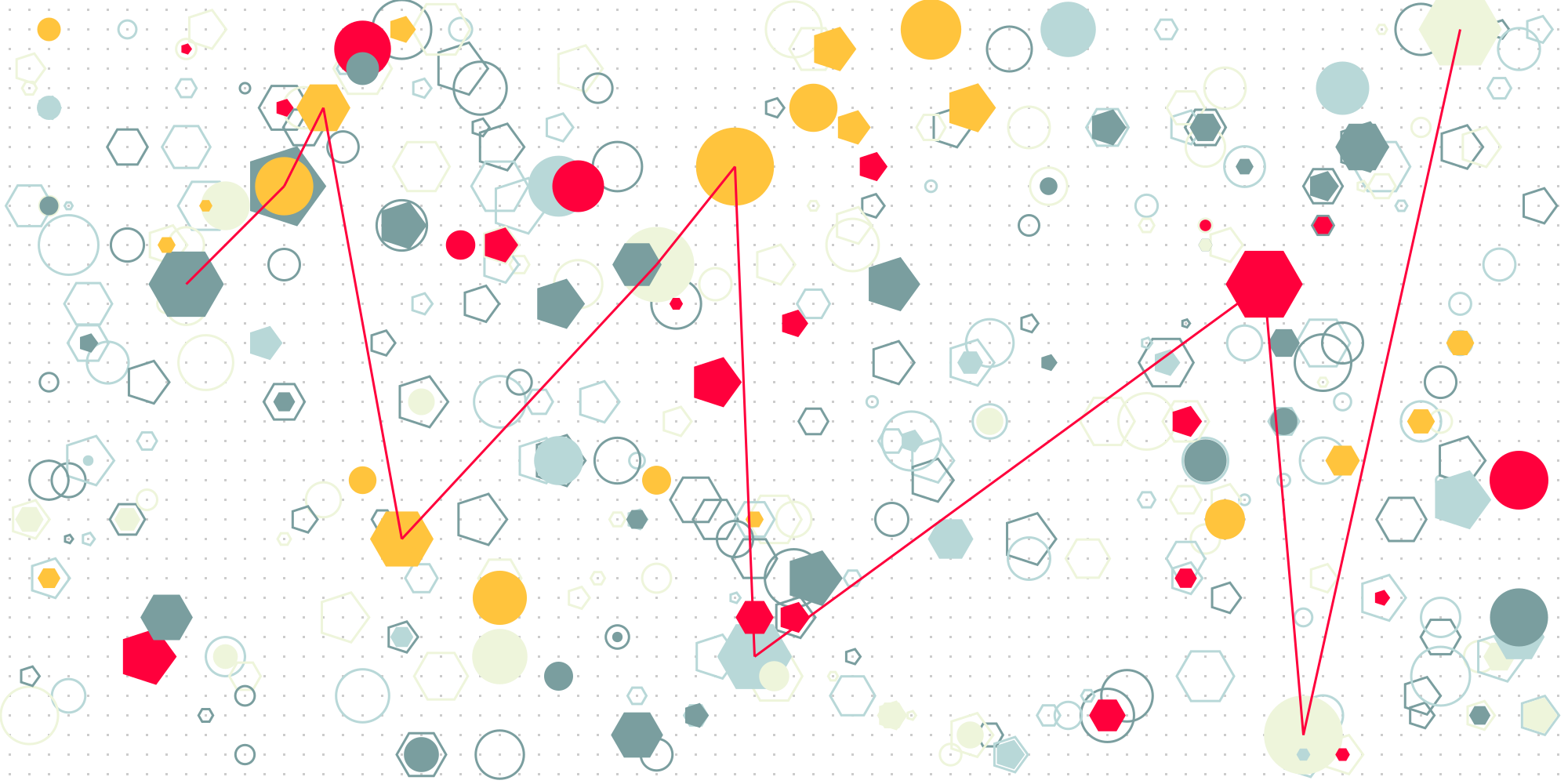
The short answer: careers that reward creative problem solving in domains with scarce knowledge. Let’s unpack that.
1 What is a Career that’s Resilient to AI?
A career resilient to AI is one in which human work remains important despite advances in AI and related technologies, such as robotics.
Such a career is interesting not because it may not involve the use of (very) advanced technologies, including AI, but because it involves solving problems, finding opportunities which technology alone cannot address, without human judgment.
2 Which Careers Are at Risk because of AI?
There have been several often mentioned publications on job or occupational exposure to artificial intelligence – see [1], [2], [3]. A common observation is that some jobs are more exposed than others, leading to the practical question of which jobs are interesting because they are less exposed. (I’m mentioning “jobs” or “occupations” here, not careers – more on this below.)
I’ll argue that while these discussions are interesting and necessary, the question can be settled in a different way – I’ll argue the following:
- Firstly, all jobs are exposed to AI and closely related technologies, such as robotics.
- Secondly, as AI will continue improving, it is more relevant to consider what properties a career should have to be resilient to AI, than how much a job is resilient, whereby a job is simply a name for work done during part of a career.
- Thirdly, careers resilient to AI are careers which involve to a significant extent the creative problem solving and development of new knowledge, or in other words, careers focused on domains for which we do not yet have well defined measures, meaning that there is little data, and consequently, any knowledge about these domains is likely going to change – basically, the only resilient careers will be those that AI cannot be applied to yet.
I’ll not discuss careers which may be resilient because they may be protected by future regulation or professional associations – those factors, if used, can be used to ensure resilience to any technology, perhaps more realistically only to postpone the impacts of technology.
3 Why Are All Jobs Exposed to AI?
AI exposure is measured by comparing the capabilities of AI with the capabilities needed in each job, following following some standard taxonomy of work; here, I’m citing research which uses the O*NET classification [6].
“To construct our measure, we identify a set of common and well-developed applications of AI based on categories defined and described by the Electronic Frontier Foundation (EFF) AI Progress Measurement project and connect these applications of AI to data on occupational abilities from the Occupational Information Network (O*NET) database developed by the United States Department of Labor.” [1]
Although the EFF AI Progress Measurement is now archived [4], current commercially available LLM-based AI systems today exhibit the same capabilities and more, and with more sophistication – for a particularly advanced case, see, e.g., [5].
Comparisons lead to observations such as the following.
“Just as with the ranked occupations, we find that the most exposed industries tend to be white collar industries requiring high levels of education, such as financial services, accounting, insurance, and legal services. Perhaps not surprisingly, exposure to AI is particularly high in certain service industries that involve a high level of information processing. On the other hand, the lowest-scoring industries tend to be blue-collar industries that involve manual labor, such as support activities for crop production, building and dwellings services, construction contracting services, and warehousing and storage.” [1]
A similar conclusion is given elsewhere [2].
“We found in our analysis that about half of workers (52%) in the professional, scientific and technical services sector face a high degree of exposure to AI, more than double the rate for workers overall. Exposure is also much greater than average for workers in finance, insurance and real estate (37%) and public administration (36%). At the other end of the spectrum, about half of workers (48%) in the other services sector, including repair, maintenance, personal and household services, are likely to experience little exposure to AI. More than four-in-ten workers in managerial and administrative services (45%) and accommodation and food services (43%) should see relatively minimal exposure to AI. These are sectors in which workers, like nannies, are more likely to engage in physical or social tasks.” [2]
This seems to suggest that some jobs are more and some less exposed to AI. An important conclusion is that information processing jobs are likely to be the most exposed ones.
“Intuitively, these occupation and industry rankings reflect (1) our aim to isolate the exposure to advances in AI (as opposed to, say, robotics, machine vision, autonomous guided vehicles, or other types of advanced technologies), and (2) the abilities most likely to be affected by AI. Whereas robotic technologies often involve physical manipulation and are capable of performing complex manual tasks, AI technologies are largely software-based and rely on iterative learning and perception (Raj & Seamans, 2019). Accordingly, we expect AI to have a limited influence on the role of physical abilities in occupations and industries. Instead, our methodology leads us to believe that AI is likely to have the biggest impact on abilities related to information processing.” [1]
AI and robotics are complementary technologies, in particular because the latter cannot really do much without the former. Consequently, there is no clear reason why AI and robotics would not progress together, and if so, then complex manual tasks are not shielded from exposure from AI and robotics. In other words, all jobs no longer differ much – sooner or later, all jobs will be more exposed to AI, with or without robotics.
4 Careers, not Jobs
If you’ve been in a management position in any industry that was subject to technological change, or where you were part of bringing these about, it is likely that you witnessed jobs becoming obsolete, with people who did them needing to receive training so that they can move to new jobs.
Job and occupation are synonyms, while career is slightly different. It emphasizes longevity: career is defined as an occupation undertaken for a significant period of a person’s life and with opportunities for progress.
In absence of technology or other change that makes a job obsolete, there is not much difference between a job and a career. If this isn’t the case, one’s actual career will involve shifts between jobs involving different knowledge and skills, rather than the level of competence in a knowledge domain.
This makes it more efficient, with regards to planning and developing a career, to focus on what makes a career more resilient to AI, rather than what makes a job resilient.
5 Maturity of Domain Knowledge Influences a Career’s Resilience to AI
Consider again the occupations evaluated as highly exposed to AI. These are, according to [2], the following: careers in information and technology, professional, scientific and technical services, government, public administration, and military, banking, finance, accounting, real estate.
There are some common themes above, as all of them involve at least the following:
- Work involves management of information
- Progress in these domains is accompanied by structuring and organizing information better
- All jobs involve the use of knowledge that is more or less standardized and available
Simply put, an important common theme in these jobs is that there is a lot of data that represents inputs and outputs of human work, and that work involves the application of, often many rules and guidelines, in other words, there are patterns in inputs and outputs.
Consequently, either AI can be built by training from data from work (whereby the AI infers rules from data), or it can be built by eliciting and implementing rules applied through work (so that AI functions by applying rules specified by people).
In either case, the irony is that the more people invest in structuring their problem / knowledge domain, the more the reasoning and decision making in that domain will be exposed to AI.
The more mature the domain knowledge, the more exposed it is and will be to AI and related technologies.
6 Resilient Careers Involve Creative Problem Solving
Careers resilient to AI are careers careers that reward creative problem solving in domains with scarce knowledge. They involve to a significant extent the development of new knowledge, or work to get knowledge to the point that it is sufficiently structured for AI to learn from it.
The challenge, then, is how to enable a greater proportion of the working population to have such careers. Another challenge is that in each such domain, there will be strong incentives to structure knowledge, keep it confidential, and build AI that then delivers it at scale, in which case very few capture substantial value. The shorter that cycle from identifying an unstructured knowledge area, to maturing it for AI to apply, the more pressure is created to identify such domains – that is, pressure in a career to be capable both of coming up with useful and significantly new ideas, and being able to structure the knowledge that these ideas lead to.
References
- Felten, Edward, Manav Raj, and Robert Seamans. “Occupational, industry, and geographic exposure to artificial intelligence: A novel dataset and its potential uses.” Strategic Management Journal 42.12 (2021): 2195-2217. https://onlinelibrary.wiley.com/doi/full/10.1002/smj.3286
- Kochhar, R., 2023. Which U.S. Workers Are More Exposed to AI on Their Jobs?, Pew Research Center. https://www.pewresearch.org/social-trends/2023/07/26/which-u-s-workers-are-more-exposed-to-ai-on-their-jobs/
- Cazzaniga, Mauro, et al. “Gen-AI: Artificial Intelligence and the Future of Work.” International Monetary Fund Staff Discussion Notes 2024.001 (2024). https://www.imf.org/en/Publications/Staff-Discussion-Notes/Issues/2024/01/14/Gen-AI-Artificial-Intelligence-and-the-Future-of-Work-542379
- Electronic Frontier Foundation, Measuring the Progress of AI Research, https://www.eff.org/ai/metrics
- Trinh, Trieu H., et al. “Solving olympiad geometry without human demonstrations.” Nature 625.7995 (2024): 476-482. https://www.nature.com/articles/s41586-023-06747-5
- https://www.onetonline.org