Individual Decision-Making: Common Models in Economics
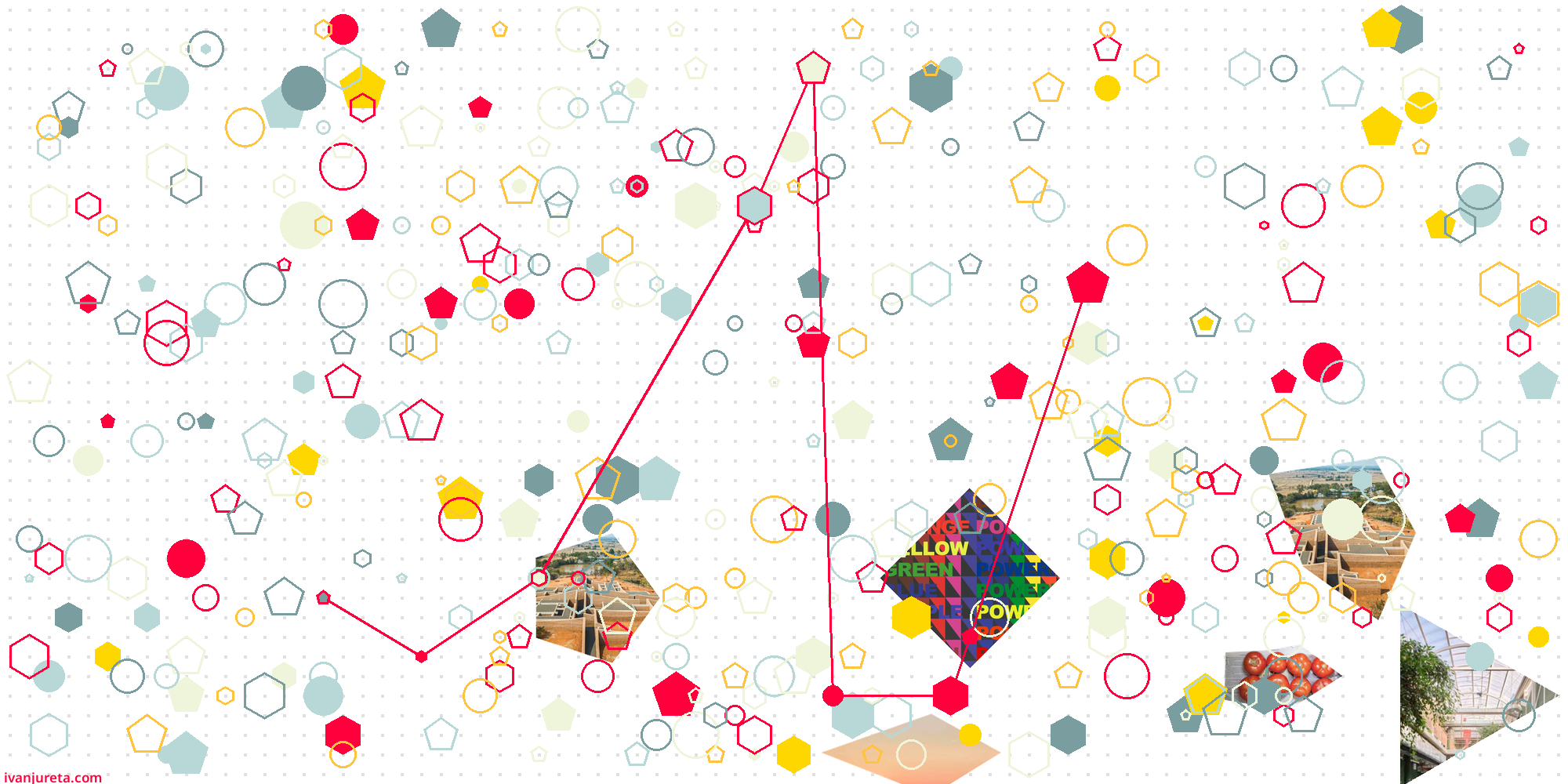
Decision-making models in economics are simplified descriptions of how decisions are made. Understanding these models is useful for designing decision governance. Decision governance will be designed in a different way depending on assumptions made about the behavior of the decision maker, the information available to them, and how the decision maker uses that information. Governance will be different if it is assumed that the decision maker follows classical utility, or expected utility, or another model. This text provides an overview of key individual decision-making models, including the classical utility model, the expected utility model, Herbert Simon’s concept of bounded rationality, Kahneman and Tversky’s behavioral models, and Gigerenzer’s heuristics-based decision-making.
This text is part of the series on decision governance. Decision Governance is concerned with how to improve the quality of decisions by changing the context, process, data, and tools (including AI) used to make decisions. Understanding decision governance empowers decision makers and decision stakeholders to improve how they make decisions with others. Start with “What is Decision Governance?” and find all texts on decision governance here.
The Classical Utility Model
The classical utility model is foundational in economics and assumes that decision-makers act to maximize utility, which represents satisfaction or benefit derived from a choice (von Neumann & Morgenstern, 1944). The model is predicated on several key assumptions:
- Rationality: Decision-makers are rational agents who consistently make choices that maximize their utility.
- Preferences: Preferences are complete and transitive, meaning decision-makers can rank all possible outcomes and their preferences remain consistent.
- Perfect Information: Decision-makers have access to all relevant information and can process it without limitations.
This model is formalized mathematically as follows: individuals choose from a set of alternatives A = {a1, a2, …, an} to maximize their utility function U(a), where U(a) represents the satisfaction derived from an alternative a. Formally, the decision-maker selects a* such that a* = argmax{U(a) | a ∈ A}. This approach relies on several axioms that connect preferences and utility:
- Completeness: For any two alternatives a and b in A, the decision-maker can state either a is preferred to b (a ≻ b), b is preferred to a (b ≻ a), or the individual is indifferent (a ∼ b).
- Transitivity: If a ≻ b and b ≻ c, then a ≻ c.
- Continuity: Preferences over outcomes are continuous, meaning that small changes in alternatives do not lead to abrupt preference reversals.
These axioms imply the existence of a utility function U(a) that assigns numerical values to alternatives, reflecting their ranking based on the decision-maker’s preferences. In this framework, utility serves as a mathematical representation of preferences, providing a structured approach to decision-making. This framework has been widely used for modeling and predicting decision-making in scenarios where individuals are assumed to have consistent preferences and full access to information. However, its limitations include oversimplifying human behavior, ignoring cognitive and informational constraints, and failing to account for psychological factors that influence real-world decisions.
The Expected Utility Model
The expected utility model, developed by John von Neumann and Oskar Morgenstern, extends the classical utility framework to situations involving uncertainty (von Neumann & Morgenstern, 1944). Decision-makers are assumed to maximize the expected value of utility, which can be calculated as:
Expected Utility (EU) = p1 ⋅ u(x1) + p2 ⋅ u(x2) + … + pn ⋅ u(xn)
where:
- p1, p2, …, pn represent the probabilities of outcomes x1, x2, …, xn,
- u(x1), u(x2), …, u(xn) are the utilities derived from these outcomes.
This model relies on several axioms of expected utility theory:
- Completeness: For any two outcomes x and y, the decision-maker can state that x is preferred to y, y is preferred to x, or they are equally preferred.
- Transitivity: If x is preferred to y, and y is preferred to z, then x must be preferred to z.
- Independence: If a decision-maker is indifferent between x and y, they will remain indifferent if both are mixed with a third outcome z in the same proportion.
- Continuity: If x is preferred to y and y is preferred to z, then there exists some probability mix between x and z such that the individual is indifferent between the mix and y.
The expected utility model ties together several key concepts:
- Utility quantifies how desirable an outcome is for a decision-maker. Higher utility values indicate more preferred outcomes.
- Preferences are the rankings of outcomes based on desirability. A preference ordering implies that if u(x1) > u(x2), outcome x1 is preferred to x2.
- Options are the set of available choices (actions or decisions) that a decision-maker can select.
- Outcomes are the results or consequences of choosing a specific option under a given set of conditions.
By assigning probabilities to outcomes based on the likelihood of each option leading to that outcome, the model integrates preferences and probabilities. The decision-maker chooses the option that maximizes their expected utility, providing a structured approach to decision-making.
Expected utility theory has been widely used in economics and finance to model decision-making under risk and uncertainty, particularly for investment and insurance scenarios. However, empirical evidence, such as the Allais paradox (Allais, 1953) and the Ellsberg paradox (Ellsberg, 1961), shows that individuals often violate these axioms, highlighting limitations in the model’s ability to describe real-world decision-making behavior.
Herbert Simon’s Concept of Bounded Rationality
Herbert Simon’s concept of bounded rationality challenges the notion of fully rational decision-making (Simon, 1955). According to Simon, individuals operate under cognitive and informational constraints, leading them to satisfice rather than optimize. Satisficing involves selecting an option that meets a threshold of acceptability rather than the optimal one. This occurs because the decision-maker may not have the required time, cognitive capacity, or all necessary information to identify the optimal option.
Bounded rationality recognizes that:
- Cognitive Limitations: Human cognitive capacity is limited, making it difficult to process and evaluate all possible alternatives.
- Limited Information: Decision-makers often lack access to complete information about all options and outcomes.
- Time Constraints: Decisions are frequently made under time pressure, further restricting the scope of analysis.
This framework has been influential in behavioral economics, emphasizing the importance of realistic assumptions about human behavior.
Kahneman and Tversky’s Behavioral Models
Daniel Kahneman and Amos Tversky’s contributions, particularly through prospect theory, have significantly advanced the understanding of decision-making under risk and uncertainty (Kahneman & Tversky, 1979; Tversky & Kahneman, 1992). Prospect theory departs from expected utility theory by incorporating psychological factors:
- Reference Dependence: Individuals evaluate outcomes relative to a reference point, often their current state, rather than in absolute terms.
- Loss Aversion: Losses are perceived as more painful than equivalent gains are pleasurable.
- Probability Weighting: People tend to overweight small probabilities and underweight large probabilities.
The value function in prospect theory is concave for gains, convex for losses, and steeper for losses, reflecting loss aversion. For example, consider an individual choosing between receiving $50 outright or a 50% chance of winning $100 and a 50% chance of winning nothing. While the expected monetary value is the same, many individuals prefer the guaranteed $50, illustrating how loss aversion and the weighting of probabilities affect decision-making. Prospect theory has also been instrumental in explaining phenomena such as framing effects, where the presentation of choices influences decision outcomes.
Gigerenzer’s Heuristics-Based Decision-Making
Gerd Gigerenzer’s work on heuristics challenges the view that cognitive limitations necessarily lead to suboptimal decisions (Gigerenzer et al., 1999). Instead, heuristics are seen as efficient rules of thumb that enable individuals to make decisions with limited information and computational resources. Common heuristics include:
- Recognition Heuristic: When faced with multiple options, individuals are more likely to choose the one they recognize. For example, when selecting between two brands of a product, a consumer may choose the brand they have heard of before, even without additional information about quality.
- Take-the-Best Heuristic: Decision-makers focus on the most important cue and ignore less relevant information. For instance, when buying a car, a buyer may prioritize fuel efficiency as the most critical factor and disregard other cues such as color or minor features.
- Fast-and-Frugal Trees: Simplified decision trees that prioritize speed and simplicity over exhaustive analysis. For example, a hiring manager may use a simple rule such as “If the candidate has five years of experience, proceed to interview; otherwise, reject,” instead of evaluating every detail of each application.
Gigerenzer argues that heuristics can lead to outcomes that are as good as, or even better than, those derived from complex optimization models, particularly in environments characterized by uncertainty and incomplete information.
Similarities and Differences
While these models differ in their assumptions and applications, they share a common goal of explaining and improving individual decision-making. However, they differ significantly in the types of information they incorporate and the ways they process it:
- Classical and Expected Utility Models: These models assume that decision-makers have access to all relevant information and the cognitive ability to process it fully. They rely on quantified preferences (utility) and probabilities to determine the optimal choice. However, they exclude psychological or situational factors and assume perfect rationality.
- Bounded Rationality: Herbert Simon’s model incorporates cognitive and informational limitations. It assumes that decision-makers operate under constraints such as limited time and incomplete information. The focus shifts from optimizing to satisficing, where individuals select an acceptable option rather than the best possible one.
- Behavioral Models (Prospect Theory): Kahneman and Tversky’s approach introduces psychological factors, such as loss aversion, reference dependence, and probability weighting. Unlike classical models, these models account for how decision-makers perceive and respond to risk and uncertainty, even when such perceptions deviate from objective probabilities.
- Heuristics-Based Models: Gigerenzer’s framework emphasizes the use of simple rules or shortcuts to make decisions in environments characterized by uncertainty and incomplete information. These models prioritize efficiency and adaptability over exhaustive information processing, contrasting with the rational optimization of classical models.
These differences show that some models are designed to represent idealized decision-makers who assume perfect information and rationality, while others incorporate observed patterns and empirical data about how people actually behave. Classical models focus on precise predictions for idealized situations, whereas behavioral and heuristics-based models seek to explain and predict real-world behavior in complex environments. Simon’s bounded rationality serves as a middle ground, emphasizing the constraints under which decisions are made.
References and Further Reading
- von Neumann, J., & Morgenstern, O. (1944). Theory of Games and Economic Behavior. Princeton University Press.
- Allais, M. (1953). “Le comportement de l’homme rationnel devant le risque: critique des postulats et axiomes de l’école américaine.” Econometrica, 21(4), 503-546.
- Ellsberg, D. (1961). “Risk, Ambiguity, and the Savage Axioms.” The Quarterly Journal of Economics, 75(4), 643-669.
- Simon, H. A. (1955). “A Behavioral Model of Rational Choice.” Quarterly Journal of Economics, 69(1), 99-118.
- Kahneman, D., & Tversky, A. (1979). “Prospect Theory: An Analysis of Decision under Risk.” Econometrica, 47(2), 263-291.
- Gigerenzer, G., Todd, P. M., & the ABC Research Group. (1999). Simple Heuristics That Make Us Smart. Oxford University Press.
- Tversky, A., & Kahneman, D. (1992). “Advances in Prospect Theory: Cumulative Representation of Uncertainty.” Journal of Risk and Uncertainty, 5(4), 297-323.
Definitions
- Utility: A measure of satisfaction or benefit derived from a choice, often expressed as a numerical value in economic models. (von Neumann & Morgenstern, 1944)
- Satisficing: A decision-making strategy that aims for a satisfactory solution rather than an optimal one. (Simon, 1955)
- Loss Aversion: The tendency to prefer avoiding losses over acquiring equivalent gains. (Kahneman & Tversky, 1979)
- Heuristic: A simple rule or strategy used to make decisions efficiently with limited information. (Gigerenzer et al., 1999)
Decision Governance
This text is part of the series on the design of decision governance. Other texts on the same topic are linked below. This list expands as I add more texts on decision governance.
- Introduction to Decision Governance
- Stakeholders of Decision Governance
- Foundations of Decision Governance
- How to Spot Decisions in the Wild?
- When Is It Useful to Reify Decisions?
- Decision Governance Is Interdisciplinary
- Individual Decision-Making: Common Models in Economics
- Group Decision-Making: Common Models in Economics
- Individual Decision-Making: Common Models in Psychology
- Group Decision-Making: Common Models in Organizational Theory
- Role of Explanations in the Design of Decision Governance
- Design of Decision Governance
- Design Parameters of Decision Governance
- Factors influencing how an individual selects and processes information in a decision situation, including which information the individual seeks and selects to use:
- Psychological factors, which are determined by the individual, including their reaction to other factors:
- Attention:
- Memory:
- Mood:
- Emotions:
- Commitment:
- Temporal Distance:
- Social Distance:
- Expectations
- Uncertainty
- Attitude:
- Values:
- Goals:
- Preferences:
- Competence
- Social factors, which are determined by relationships with others:
- Impressions of Others:
- Reputation:
- Social Hierarchies:
- Social Hierarchies: Why They Matter for Decision Governance
- Social Hierarchies: Benefits and Limitations in Decision Processes
- Social Hierarchies: How They Form and Change
- Power: Influence on Decision Making and Its Risks
- Power: Relationship to Psychological Factors in Decision Making
- Power: Sources of Legitimacy and Implications for Decision Authority
- Power: Stability and Destabilization of Legitimacy
- Power: What If High Decision Authority Is Combined With Low Power
- Power: How Can Low Power Decision Makers Be Credible?
- Social Learning:
- Psychological factors, which are determined by the individual, including their reaction to other factors:
- Factors influencing information the individual can gain access to in a decision situation, and the perception of possible actions the individual can take, and how they can perform these actions:
- Governance factors, which are rules applicable in the given decision situation:
- Incentives:
- Incentives: Components of Incentive Mechanisms
- Incentives: Example of a Common Incentive Mechanism
- Incentives: Building Out An Incentive Mechanism From Scratch
- Incentives: Negative Consequences of Incentive Mechanisms
- Crowding-Out Effect: The Wrong Incentives Erode the Right Motives
- Crowding-In Effect: The Right Incentives Amplify the Right Motives
- Rules
- Rules-in-use
- Rules-in-form
- Institutions
- Incentives:
- Technological factors, or tools which influence how information is represented and accessed, among others, and how communication can be done
- Environmental factors, or the physical environment, humans and other organisms that the individual must and can interact with
- Governance factors, which are rules applicable in the given decision situation:
- Factors influencing how an individual selects and processes information in a decision situation, including which information the individual seeks and selects to use:
- Change of Decision Governance
- Public Policy and Decision Governance:
- Compliance to Policies:
- Transformation of Decision Governance
- Mechanisms for the Change of Decision Governance