Business Risks of IP Compliance Requirements for Generative AI
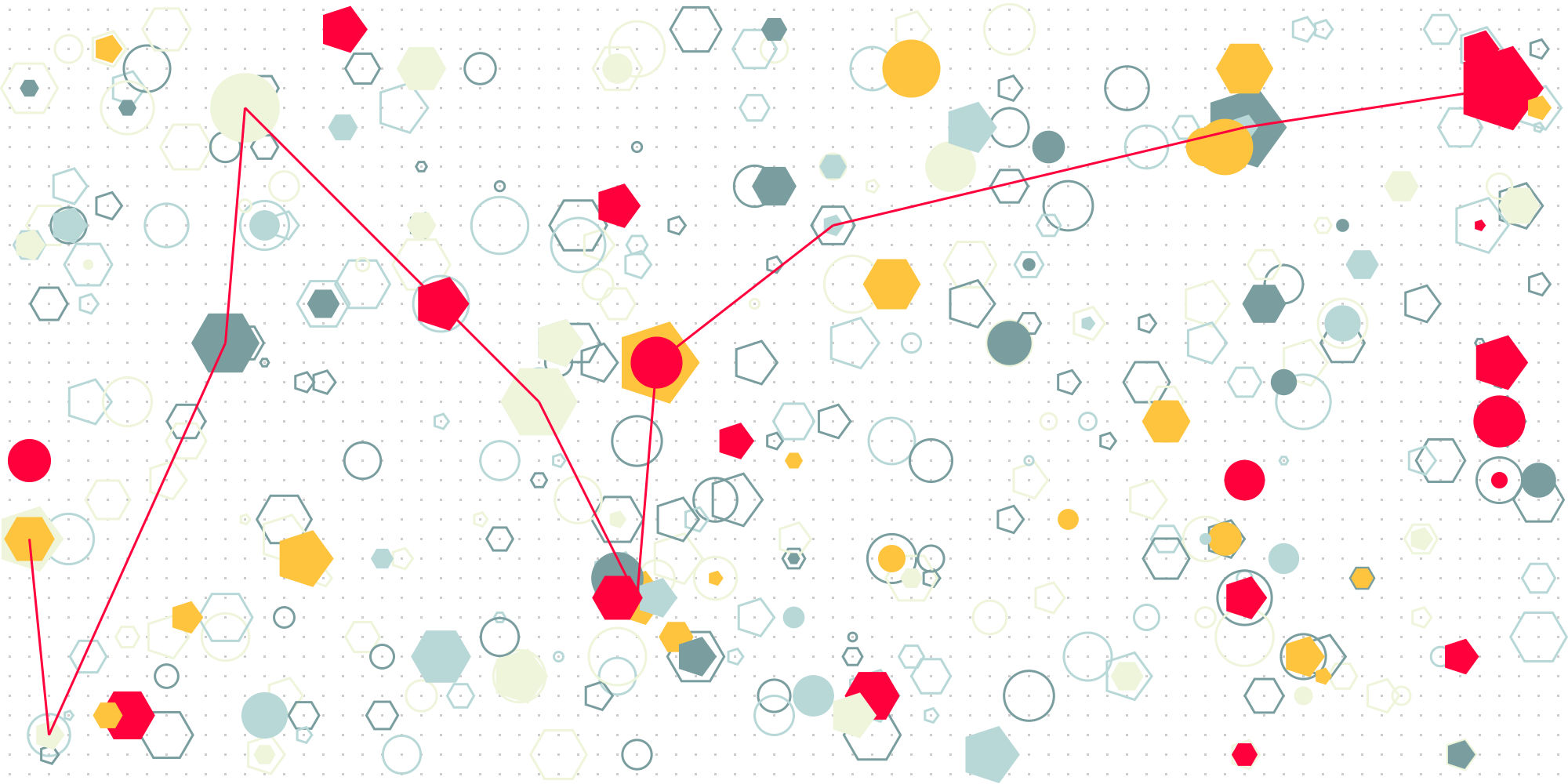
What are the requirements that a product/service should comply with, if it produces output to customers using generative AI?
Legislation provides high-level requirements, and draft legislation in China is one of the available cases. As Xiao writes, here, on April 11, 2023, “the Cyberspace Administration of China released a draft of the Regulation for Generative Artificial Intelligence Services”. Below is his summary that suggests compliance requirements:
“To avoid potential liability, providers should ensure that the pre-training and optimization training data used for generative AI products meets the following requirements:
- it complies with the requirements of laws and regulations such as the Cybersecurity Law;
- it does not contain content that infringes on IP rights;
- if the data contains personal information, the consent of the holder of the personal information shall be obtained or comply with other statutory circumstances;
- the authenticity, accuracy, objectivity, and diversity of the data can be guaranteed; and
- it complies with other regulatory requirements (Article 7).”
Leaving aside existing regulations (items 1 and 5), the new requirements concern use of existing intellectual property for training (item 2), consent to personal information use for training (item 3), and specific data quality requirements (item 4).
All compliance requirements increase barriers to entry, by increasing compliance costs, which show up both before going to market, let’s say, the product development stage, and then, cost of operating the product/service.
Simply put, any entrant will need more funding to go to market relative to, say, the funding needed to get a less regulated digital service. This is obvious, and is an observation that can be made for any industry that’s more regulated versus one that is less.
What’s different about generative AI, is (as Xiao observes as well) that compliance requirements above reduce the readily and cheaply available amount of training data, with a few consequences on how product development and product operations are done.
If some minimal threshold of training data quantity and variety is not achieved, this will reduce the quality of the trained AI, and increase risk of dissatisfied customers. Hence the need to have, during product development, experts capable of assessing the relationship between the properties of the training data and the output quality. Such experts are rare, so again, product development costs increase.
Requirement 2, on IP infringement, is a very tricky one.
Firstly, traceability of IP rights on training content that is readily available (think using web data) is hard, i.e., it is hard to establish what is subject to IP regulation, which regulation (geography matters), who the owner is, and even what that is accomplished, think of the cost of negotiating rights to use that content with millions of parties across different jurisdictions. This can be dealt with in several ways:
- Use datasets with known owners, IP licensing conditions and costs;
- Build datasets that your product/service needs;
- Use any available data and accept the risk of non-compliance; note the ethical dilemma of doing so (think of, e.g., Facebook’s handling of damaging content, Uber’s relationship to taxi operators, how late Youtube started actively dealing with copyrighted content, etc.). For those who ignore the ethical dilemma, the issue is how to grow fast to a size at which the company can absorb the cost of non-compliance, that is, reaches the point at which the cost cannot break it, but is a new tax on operations.
In the case of Hilbert Paradox SA, which I was a co-founder and Chief Scientific Officer of (it was bought by Genae, which was then bought by IQVIA), we built our datasets and used ones with clear traceability. We were using healthcare data, and running any risk around provenance and rights on training data is unacceptable.
Secondly, licenses have an end date and need to be renegotiated. An obvious consequence is that there is risk that the data you licensed is no longer available to you. This can break a business model, if that data was critical to what the product/service does. If such data was used to train the AI, the interesting question is if the AI, upon license expiry and non-renewal, needs to be retrained without that underlying data. The bigger the resources needed to train the model, the bigger the negative consequences of this risk.
As an aside, note that a dataset, to be useful, needs to be continuously updated. In other words, buying or licensing a static dataset is less relevant than licensing the data stream that produces up to date data from the underlying process that generates this data. A simple example is weather data: it is less valuable to have, say, a dataset from 1950s to exactly now, than to have a license to use that and all data collected in the future, up to license expiry.
I will discuss Requirements 2 and 3 in other notes on this site.
Clearly, how training data is sourced is a strategic question.