Decision Governance Concepts: Outcomes to Explanations
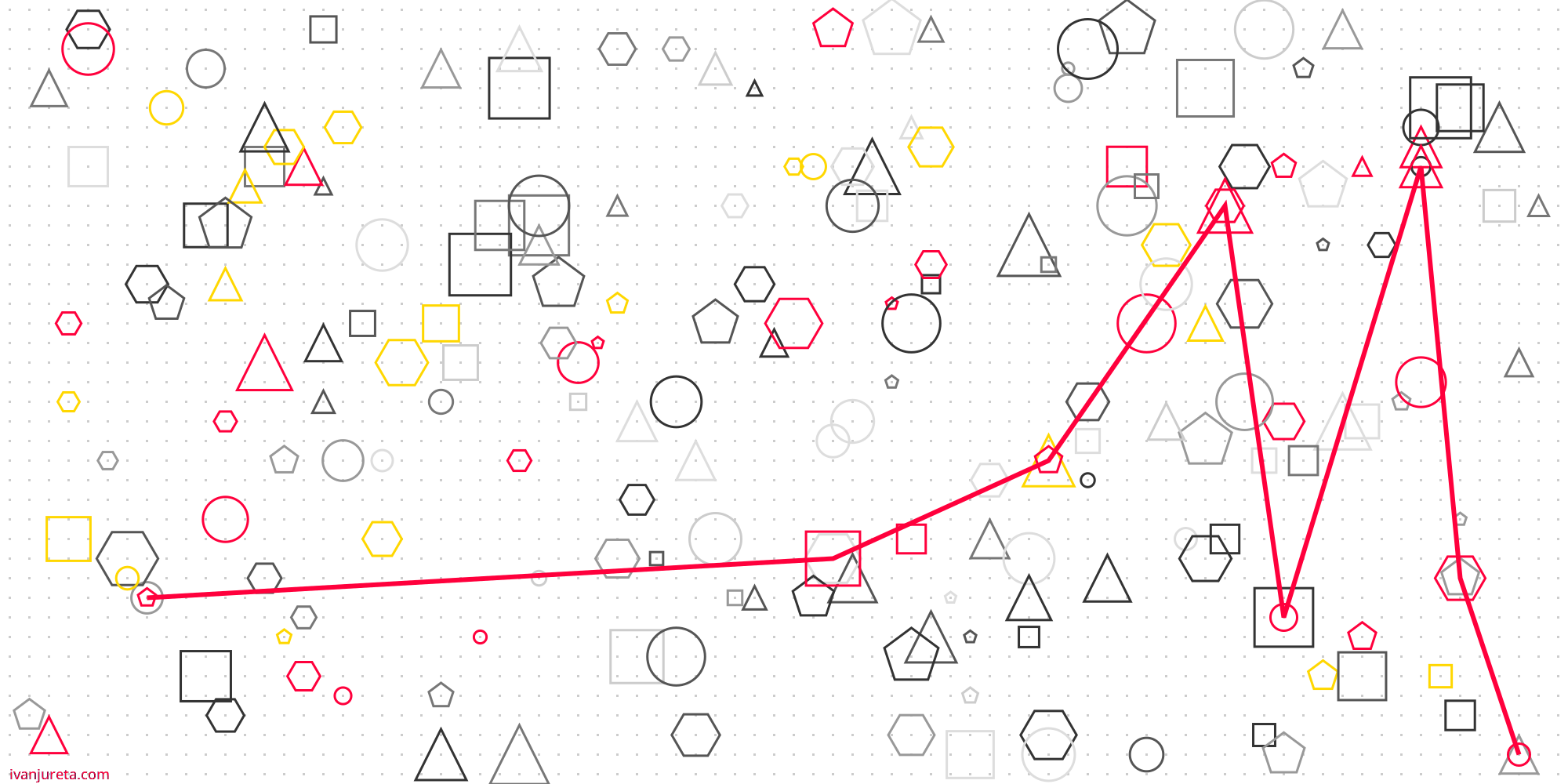
Let’s assume that there is a situation you observed, and you want to understand the decision that led to it – maybe there is something particularly good about the situation and you want to see how to increase the probability that this happens again, or there is something you would want to prevent from happening again in the future. In both cases, the aim is to go back from what you observed to the explanation of the decision, so that we can then look into how to design decision governance that would apply next time the same/similar decision needs to be made.
This text is part of the series on decision governance. Decision Governance is concerned with how to improve the quality of decisions by changing the context, process, data, and tools (including AI) used to make decisions. Understanding decision governance empowers decision makers and decision stakeholders to improve how they make decisions with others. Start with “What is Decision Governance?” and find all texts on decision governance here.
The starting point is something that you have observed or are observing still (circle labeled 1), and that is of interest to you. These outcomes are observable in some broader situation (circle 2). The situation is shown and distinguished from outcomes because it can happen, as we analyze the outcomes, that they are dependent in some way on other events or other, that are part of the situation. The boundary between the observable outcomes and the situation may not be easy to define.
For example, let’s assume a firm released a new product, and they are not seeing sales growing at the expected rate. While the sales are observable outcomes of interest, other events may have occurred, and they may not be easily separable from the slow sales – there may have been change of regulations, change in customer expectations, introduction of substitute products, poor reviews, and so on.
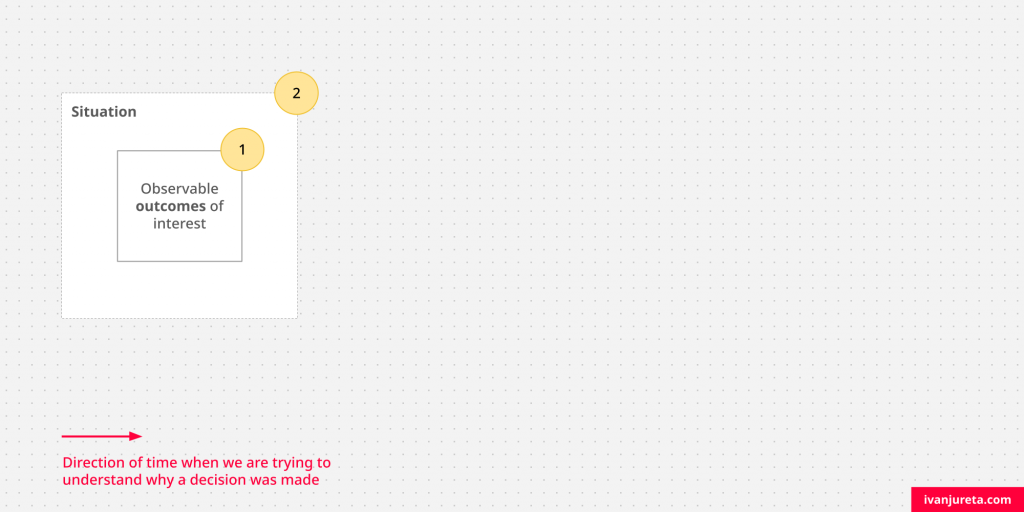
In order to change the outcomes by changing decision governance, we need to identify actions that likely led to these outcomes (circle 3). The effort required to identify actions will depend on how typical the outcomes are, and how well causes of them may be. The more typical, the more likely there are documented processes to produce them, and so looking at process definitions will be useful. At other times, when the outcomes are rare, investigations such as root cause analysis will be useful.
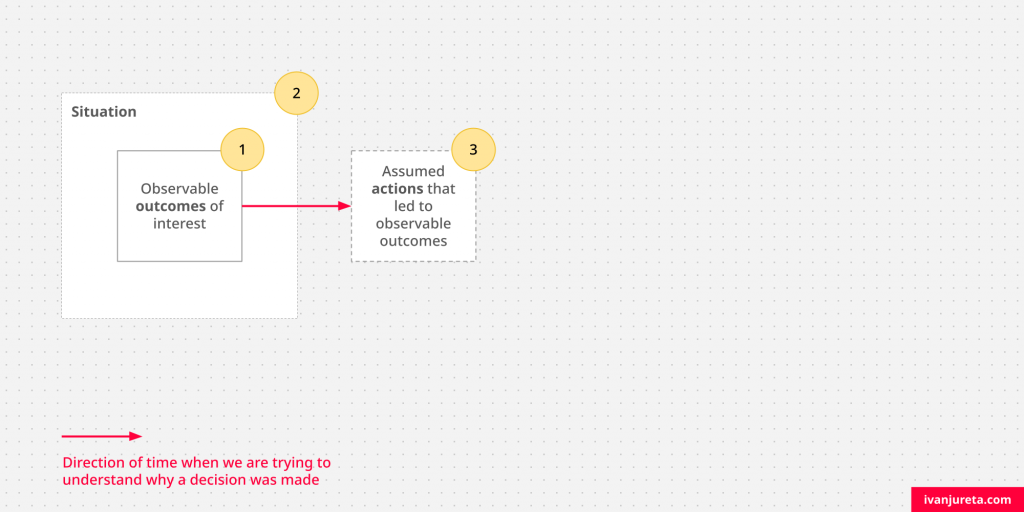
Continuing the example of slow product sales, there are many actions which could have led to that outcome. The product may not meet the specific needs or preferences of the target market. This could result from incorrect assumptions about customer problems or expectations, leading to a mismatch between what is offered and what is needed. The design may have further been deficient, in that it failed to lead to a product that differentiates from others targeting the same needs. If customers cannot see a clear reason to choose the product over competing offerings, sales will likely underperform. Pricing that is either too high (relative to perceived value) or too low (creating the impression of low quality) can result in poor sales. For example, premium pricing without corresponding product benefits can drive customers to seek alternatives.
The actions were taken because there had to be decisions leading to them (circle 4).
In the example, the wrong price may have been decided for the launch, and prior to that, wrong design decisions may have been made.
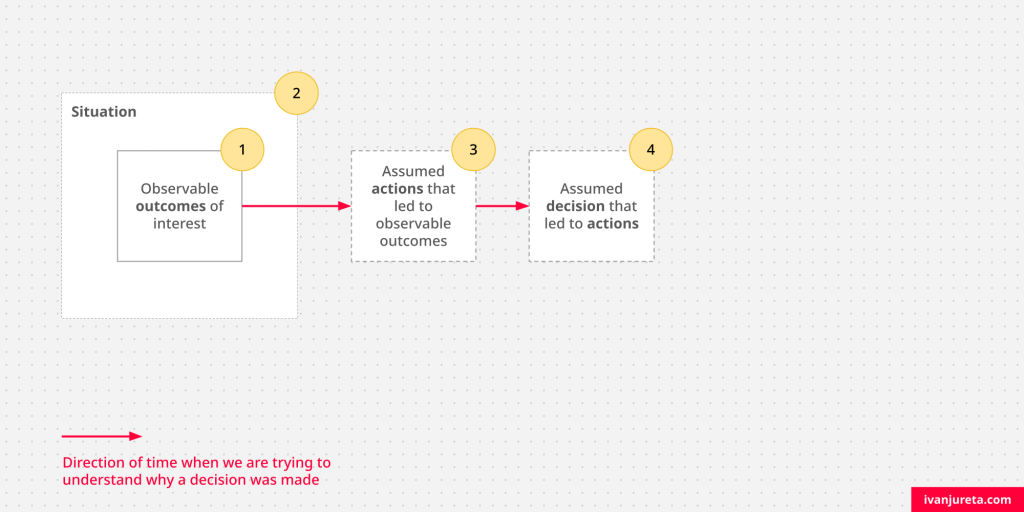
If decisions were taken, then there may be records of them (circle 5). This is not necessarily the case, but if found, records of the decision can help identify who was involved in making the decision, what their roles were, and information used to make the decision; they may point to applicable standards, rules, obligations, or other factors that influenced the decision.
In the example, various kinds of records, or evidence can suggest poor decisions: documented pricing strategy, documented design rationale, records of market research data and analysis thereof, and others.
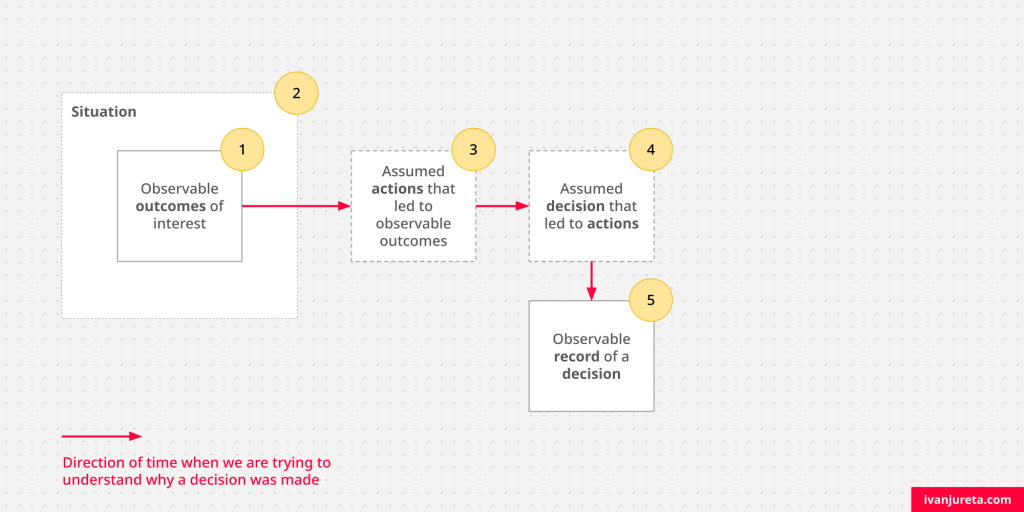
Given that there was a decision, that someone chose to have the actions taken, we need to also assume that there are reasons that decision was made. This leads us to assume that there is an explanation for the decision (circle 6). The reason it is interesting to assume that there is an explanation for a decision, is that there can be various kinds of reasons, more or less well structured, and more or less well founded, that could have led to the decision.
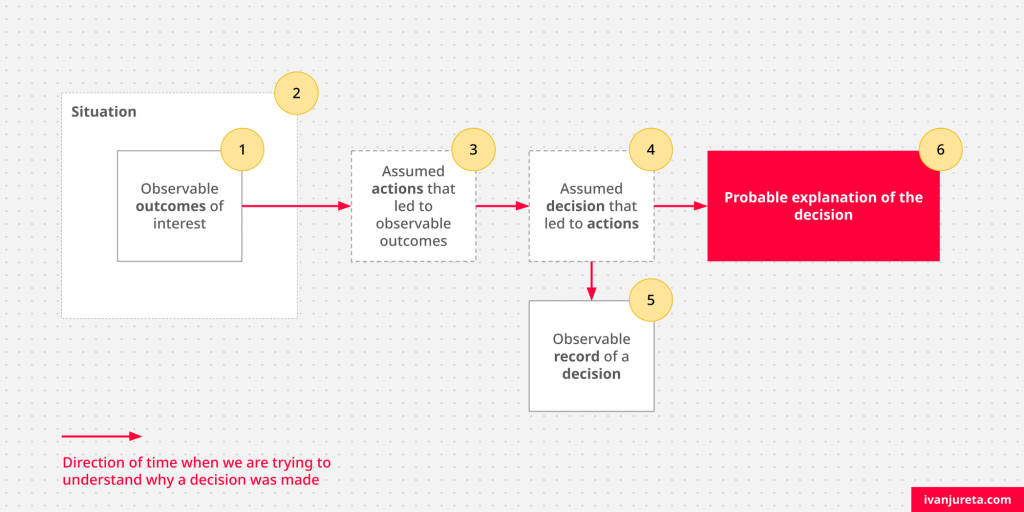
We might think that any decision should be explainable as a result of a rational process, in which many options were considered, compared using some criteria, and so on – but that is one type of explanation we could provide, but is not the only one. A decision may have been a result of deliberation between very few options. It could have been a result of no deliberation at all, but may have been forced on the decision maker. And so on – point being that explanations for decisions can be structured in various ways, and we don’t need to make any assumptions about this yet; texts after this one will deal with what kinds of explanations could be provided, and their relative merits and limitations.
In the example, here are three alternative explanations – all of which can be described by different decision making models (decision analysis, models based on heuristics, argumentation models, and so on).
- Poor Decision Making Driven by Emotions and Attitudes: In this scenario, the failure could result from a leader’s emotional attachment to the product or an overly optimistic attitude. For example, one might push a product to market because of personal enthusiasm or ego, believing it aligns with their vision or status within the industry. This emotional bias can lead to ignoring market research or dismissing critical feedback from team members. Emotional attachment often clouds objective evaluation, causing decision-makers to overlook flaws in product design or market positioning, resulting in overconfidence and misjudging customer demand. This emotional-driven approach might manifest as the “sunk cost fallacy,” where the decision-maker continues to invest in a failing product because of the resources already committed, rather than rationally cutting losses.
- Rational Decision Making with Poor Data: Here, the decision-maker operates in a rational manner but is hindered by inaccurate, incomplete, or outdated data. For instance, a product may be developed and launched based on market research that misrepresents consumer preferences due to small sample sizes, skewed surveys, or changes in market conditions that were not reflected in the data used. If decision-makers rely on faulty data to determine pricing, features, or target demographics, their rational strategies will still lead to poor outcomes. In this case, the firm could have made logical, well-reasoned decisions based on cost-benefit analysis, but the inputs into that analysis—data on market size, customer behavior, or competitive positioning—were flawed, leading to product underperformance.
- Groupthink Leading to Inadequate Decisions: Groupthink occurs when a cohesive decision-making group prioritizes consensus and harmony over critical evaluation, leading to suboptimal outcomes. In this situation, the leadership team or project group responsible for the product development might have fallen into groupthink, where dissenting opinions or concerns about the product’s viability were either suppressed or not raised at all. Group members may have been reluctant to challenge the dominant view, fearing conflict or believing that unanimity signified sound decision-making. For example, if the senior leadership team unanimously agreed to fast-track the product launch based on collective overconfidence or a shared desire to meet an ambitious deadline, they may have overlooked important risks or ignored potential weaknesses in the product’s design. The pressure for consensus can inhibit critical thinking, resulting in the team collectively making decisions that fail to properly address market challenges or product deficiencies.
While we can assume that there is an explanation for a decision, the problem then is to know what the right explanation is, before we decide how to govern such decision situations going forward.
Decision Governance
This text is part of the series on the design of decision governance. Other texts on the same topic are linked below. This list expands as I add more texts on decision governance.
- Introduction to Decision Governance
- Stakeholders of Decision Governance
- Foundations of Decision Governance
- How to Spot Decisions in the Wild?
- When Is It Useful to Reify Decisions?
- Decision Governance Is Interdisciplinary
- Individual Decision-Making: Common Models in Economics
- Group Decision-Making: Common Models in Economics
- Individual Decision-Making: Common Models in Psychology
- Group Decision-Making: Common Models in Organizational Theory
- Role of Explanations in the Design of Decision Governance
- Design of Decision Governance
- Design Parameters of Decision Governance
- Factors influencing how an individual selects and processes information in a decision situation, including which information the individual seeks and selects to use:
- Psychological factors, which are determined by the individual, including their reaction to other factors:
- Attention:
- Memory:
- Mood:
- Emotions:
- Commitment:
- Temporal Distance:
- Social Distance:
- Expectations
- Uncertainty
- Attitude:
- Values:
- Goals:
- Preferences:
- Competence
- Social factors, which are determined by relationships with others:
- Impressions of Others:
- Reputation:
- Promises:
- Social Hierarchies:
- Social Hierarchies: Why They Matter for Decision Governance
- Social Hierarchies: Benefits and Limitations in Decision Processes
- Social Hierarchies: How They Form and Change
- Power: Influence on Decision Making and Its Risks
- Power: Relationship to Psychological Factors in Decision Making
- Power: Sources of Legitimacy and Implications for Decision Authority
- Power: Stability and Destabilization of Legitimacy
- Power: What If High Decision Authority Is Combined With Low Power
- Power: How Can Low Power Decision Makers Be Credible?
- Social Learning:
- Psychological factors, which are determined by the individual, including their reaction to other factors:
- Factors influencing information the individual can gain access to in a decision situation, and the perception of possible actions the individual can take, and how they can perform these actions:
- Governance factors, which are rules applicable in the given decision situation:
- Incentives:
- Incentives: Components of Incentive Mechanisms
- Incentives: Example of a Common Incentive Mechanism
- Incentives: Building Out An Incentive Mechanism From Scratch
- Incentives: Negative Consequences of Incentive Mechanisms
- Crowding-Out Effect: The Wrong Incentives Erode the Right Motives
- Crowding-In Effect: The Right Incentives Amplify the Right Motives
- Rules
- Rules-in-use
- Rules-in-form
- Institutions
- Incentives:
- Technological factors, or tools which influence how information is represented and accessed, among others, and how communication can be done
- Environmental factors, or the physical environment, humans and other organisms that the individual must and can interact with
- Governance factors, which are rules applicable in the given decision situation:
- Factors influencing how an individual selects and processes information in a decision situation, including which information the individual seeks and selects to use:
- Change of Decision Governance
- Public Policy and Decision Governance:
- Compliance to Policies:
- Transformation of Decision Governance
- Mechanisms for the Change of Decision Governance