Decision Governance Is Interdisciplinary
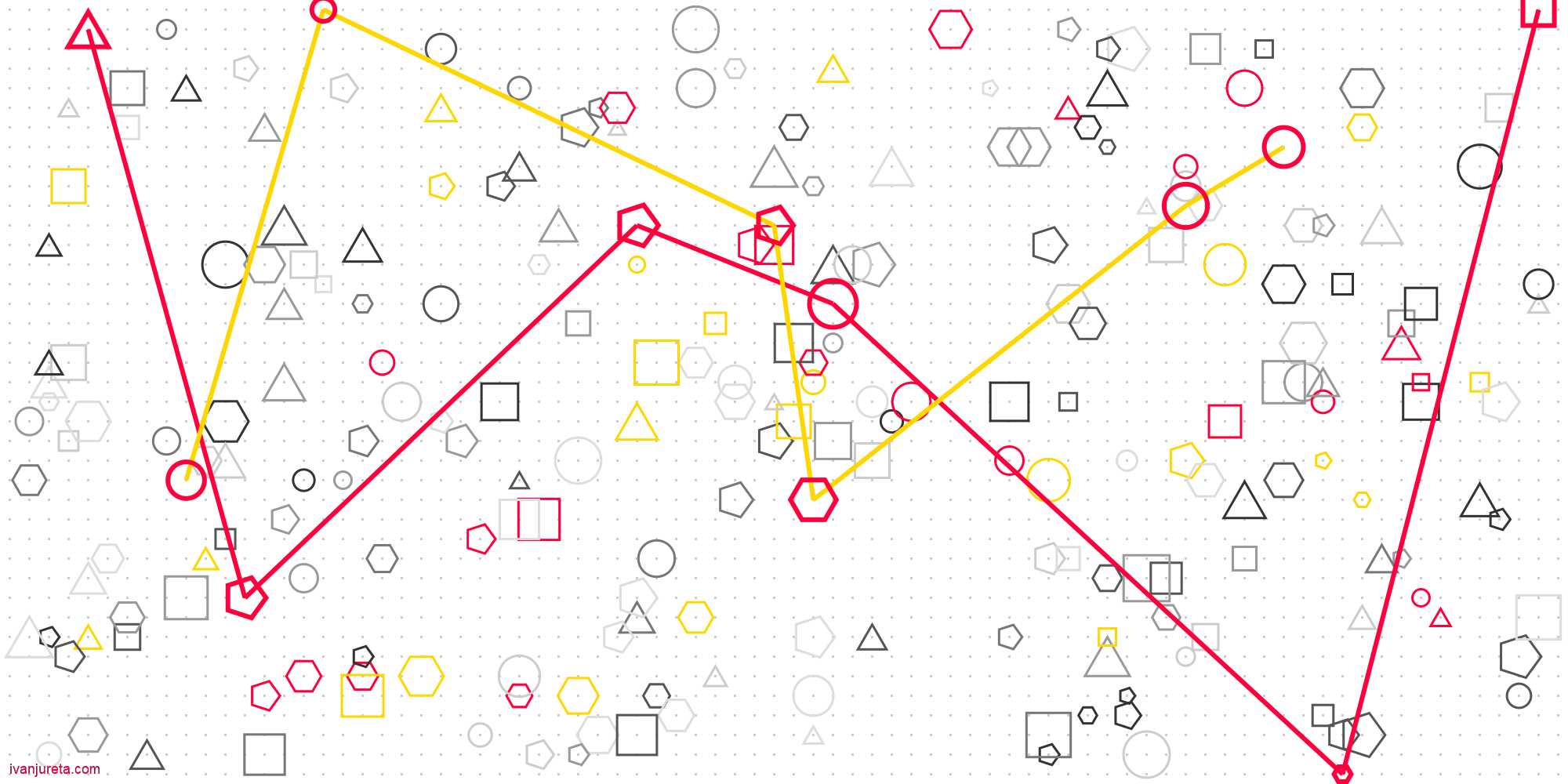
Decision governance, as a structured approach to improving the quality of decision-making, draws on a diverse array of academic disciplines. Understanding these areas can help in the design of frameworks for specific organizational, economic, and social environments. This text is a light walkthrough of key research domains relevant to decision governance.
This text is part of the series on the design of decision governance. Decision Governance refers to values, principles, practices designed to improve the quality of decisions. Find all texts on decision governance here, including “What is Decision Governance?” here.
Behavioral Economics
Behavioral economics studies how psychological, social, and emotional factors influence decision-making. Insights from this field highlight systematic biases, such as overconfidence, loss aversion, and anchoring, which often distort judgments (Kahneman, 2011). Decision governance can leverage behavioral economics to design interventions, such as choice architecture, that guide decision-makers toward more rational outcomes. For example, nudging—a concept popularized by Thaler and Sunstein (2008)—can be applied to frame options in a way that aligns individual choices with organizational objectives.
Choice architecture involves structuring the decision-making environment to influence outcomes without restricting options. This can include strategies such as default settings, which leverage individuals’ tendency to stick with pre-selected choices, or simplifying complex information to make choices easier to evaluate. For instance, automatically enrolling employees in retirement savings plans with an option to opt out has been shown to significantly increase participation rates (Thaler & Sunstein, 2008). Similarly, presenting information in a way that highlights key trade-offs—such as showing the health impacts of various food options—can steer decisions toward more beneficial outcomes. Effective choice architecture integrates insights about human behavior to design decision environments that enhance both individual and organizational objectives.
Behavioral economics also emphasizes the importance of feedback loops in decision-making. Studies show that timely, accurate feedback can mitigate cognitive biases and improve decision quality over time (Kahneman, 2011). Governance systems should integrate mechanisms for collecting and disseminating feedback, ensuring continuous learning.
Organizational Theory
Organizational theory examines how structures, cultures, and processes influence behavior within organizations (Simon, 1947). Decision governance benefits from this field by adopting principles that ensure alignment between decision-making processes and organizational goals. For instance, the concept of decentralized decision-making, explored extensively in organizational theory, can be applied to empower teams closest to specific problems while maintaining accountability.
Contemporary research expands on this idea by emphasizing dynamic capabilities—the ability of an organization to adapt to changing environments—as a critical factor in effective decision-making (Teece, 1997). Dynamic capabilities enable organizations to reconfigure their resources and processes in response to external shifts, ensuring resilience and adaptability.
Another modern contribution comes from studies on ambidextrous organizations, which focus on balancing exploration and exploitation to achieve both innovation and operational efficiency (O’Reilly & Tushman, 2004). Decision governance frameworks informed by this perspective can help organizations allocate resources effectively across these competing priorities, ensuring that short-term and long-term objectives are addressed simultaneously.
Power dynamics also remain a critical consideration in organizational theory (Simon, 1947). Effective governance frameworks account for these dynamics, ensuring that authority is balanced with transparency and that diverse perspectives are included to mitigate risks of groupthink. Incorporating insights from contemporary studies, governance systems should also facilitate psychological safety—a climate where individuals feel comfortable sharing their views—to promote innovation and better decision outcomes.
Systems Thinking
Systems thinking provides tools for understanding and managing complexity by focusing on the interrelationships within a system rather than isolated components (Sterman, 2000). This approach is particularly valuable in decision governance, where decisions often have cascading effects. Systems thinking encourages decision-makers to map dependencies, feedback loops, and potential unintended consequences.
A key concept in systems thinking is the use of causal loop diagrams to visualize the feedback mechanisms that can either stabilize or destabilize a system (Senge, 1990). These diagrams help decision-makers identify leverage points where interventions can produce significant changes. For example, reinforcing feedback loops can amplify successes, while balancing loops can mitigate risks.
Scenario analysis is another critical tool derived from systems thinking. This method involves simulating the outcomes of different decision pathways under varying conditions, allowing organizations to anticipate risks and opportunities (Sterman, 2000). By embedding scenario analysis in governance frameworks, decision-makers can test assumptions and plan for contingencies.
Systems thinking also emphasizes the need for holistic performance metrics that capture the interconnected impacts of decisions across organizational units and external stakeholders. For instance, a supply chain optimization decision must consider not only cost savings but also environmental and social implications, ensuring alignment with broader organizational values (Senge, 1990).
Decision Sciences
Decision sciences encompass quantitative and qualitative methods for analyzing decision-making processes. Techniques such as decision trees, Bayesian analysis, and multi-criteria decision analysis (MCDA) provide structured approaches to evaluating options and outcomes. These tools can be embedded into governance frameworks to standardize the evaluation process (Keeney & Raiffa, 1993).
MCDA, in particular, is widely used to address complex decisions involving multiple objectives and stakeholders. For instance, in public health decisions, MCDA can weigh trade-offs between cost, accessibility, and patient outcomes, ensuring a balanced and transparent process (Belton & Stewart, 2002). Incorporating such methodologies into governance systems ensures that decisions are data-driven and aligned with strategic priorities.
Advances in computational decision support systems have further enhanced the application of decision sciences. Tools like Monte Carlo simulations and artificial intelligence (AI)-based optimization algorithms enable decision-makers to model uncertainties and identify optimal strategies under varying conditions (Clemen & Reilly, 2013). Governance frameworks should integrate these technologies to improve decision accuracy and efficiency.
Ethics and Philosophy
Ethics provides a foundation for evaluating the fairness and legitimacy of decisions. Decision governance frameworks should incorporate ethical principles to ensure that decisions align with organizational values and societal expectations. Normative ethics, which focuses on how decisions ought to be made, offers guidelines for balancing competing interests and addressing moral dilemmas.
The concept of procedural justice, which emphasizes transparency and stakeholder participation in decision-making, is particularly relevant. Studies show that perceptions of fairness in the decision-making process significantly influence stakeholder acceptance and trust (Rawls, 1971). Governance systems should therefore establish clear processes for involving affected parties and explaining decision rationales.
Philosophical insights into epistemology—the study of knowledge—further strengthen decision governance. Epistemological frameworks help organizations assess the reliability of information sources, identify biases in evidence interpretation, and refine the assumptions underpinning their decisions (Rescher, 2003). This ensures that governance frameworks are not only effective but also defensible under scrutiny.
Data Science and Analytics
Data science plays a critical role in decision governance by providing the tools to analyze large datasets and extract actionable insights. Techniques such as predictive modeling, machine learning, and data visualization enable organizations to make evidence-based decisions. Governance frameworks should ensure that data is collected, analyzed, and presented in a way that is transparent and accessible to stakeholders.
Data science also facilitates real-time monitoring of decision outcomes, allowing organizations to adjust strategies dynamically. For example, dashboards powered by AI algorithms can provide decision-makers with continuous updates on key performance indicators (KPI), highlighting areas that require attention (Provost & Fawcett, 2013).
However, challenges such as data quality, bias, and privacy concerns must be addressed. Poor-quality data can lead to flawed decisions, while biased algorithms may reinforce existing inequities. Ethical guidelines for data usage and robust validation protocols should therefore be integral to decision governance systems (O’Neil, 2016). Additionally, fostering data literacy across organizational levels ensures that stakeholders can critically evaluate the information underpinning their decisions.
Public Policy and Political Science
Public policy research offers insights into decision-making in complex, multi-stakeholder environments. Concepts such as policy cycles, stakeholder analysis, and regulatory impact assessments are directly applicable to decision governance (Sterman, 2000). These tools help organizations anticipate external influences, such as regulatory changes or public opinion, and adapt their decision-making processes accordingly.
Political science contributes by exploring power structures, negotiation strategies, and coalition-building. Decision governance frameworks can incorporate these insights to manage conflicts of interest and foster collaboration among diverse stakeholders. For example, game theory, a tool from political science, provides frameworks for analyzing strategic interactions where the outcomes depend on the actions of multiple actors (Axelrod, 1984).
Public policy frameworks also emphasize the importance of equity in decision-making. Governance systems should evaluate how decisions impact different demographic groups, ensuring that outcomes are inclusive and socially responsible (Stone, 2002). By integrating these principles, organizations can align their decision-making processes with broader societal goals.
Cognitive Psychology
Cognitive psychology investigates the mental processes underlying decision-making, including perception, memory, and reasoning. This field sheds light on how individuals process information and the cognitive limitations that affect their judgments (Kahneman, 2011). Decision governance can use these findings to design training programs and decision-support tools that enhance cognitive performance.
For example, research on decision fatigue—the deterioration of decision quality after making many decisions—suggests that governance frameworks should limit decision-makers’ cognitive load by automating routine choices and prioritizing critical ones (Baumeister & Tierney, 2011). Cognitive load theory further recommends structuring information presentation to reduce mental effort, improving comprehension and retention (Sweller, 1988).
Cognitive psychology also highlights the role of heuristics—mental shortcuts used for decision-making. While heuristics can simplify complex problems, they can also introduce biases. Governance systems should therefore provide tools to identify and mitigate these biases, such as checklists for evaluating assumptions and alternative perspectives (Tversky & Kahneman, 1974). Incorporating such tools ensures that decisions are both efficient and well-informed.
Operations Research
Operations research focuses on optimizing decision-making through mathematical modeling and analytical methods. Tools such as linear programming, simulation, and optimization algorithms are valuable for solving complex problems with multiple constraints. Decision governance can integrate these techniques to improve resource allocation, risk management, and process efficiency.
One of the most impactful applications of operations research is in supply chain management, where models optimize trade-offs between cost, quality, and delivery time (Chopra & Meindl, 2016). These principles can also be applied to human resource planning, financial forecasting, and facility location decisions.
Emerging areas such as stochastic programming and robust optimization extend traditional operations research techniques to account for uncertainty, making them particularly useful in volatile environments (Bertsimas et al., 2011). By embedding these advanced methodologies into governance frameworks, organizations can enhance their capacity to navigate complexity and uncertainty.
Sociology and Cultural Studies
Sociology provides insights into how social norms, group dynamics, and cultural values influence decision-making. Cultural studies further explore how these factors vary across different contexts. Decision governance frameworks must account for these variations to ensure relevance and effectiveness in diverse environments (Hofstede, 2001).
Hofstede’s cultural dimensions theory, for example, highlights differences in individualism, power distance, and uncertainty avoidance across cultures. These insights can guide the design of governance systems that are culturally sensitive and adaptable (Hofstede, 2001). For multinational organizations, understanding such cultural differences is essential for ensuring that decisions are implemented effectively across regions.
Sociological research also emphasizes the importance of social capital—the networks and relationships that facilitate cooperation and trust within organizations (Putnam, 2000). Decision governance systems should foster environments that build and sustain social capital, enabling collaborative and efficient decision-making processes.
Integrative Approaches
While each of these disciplines offers unique contributions, decision governance benefits most from integrative approaches that combine insights from multiple fields. For example, behavioral economics and cognitive psychology can jointly inform strategies to address biases, while data science and decision sciences can collaborate to enhance analytical rigor. The integration of ethical principles ensures that these technical solutions align with broader organizational values.
Designing effective decision governance systems requires drawing on a wide range of academic disciplines, each of which provides valuable insights into different aspects of decision-making. By integrating principles from behavioral economics, organizational theory, systems thinking, decision sciences, ethics, data science, public policy, cognitive psychology, operations research, and sociology, organizations can create frameworks that are robust, adaptive, and aligned with their goals. Understanding these disciplines and their interplay is essential for improving decision quality.
References and Further Reading
- Thaler, R. H., & Sunstein, C. R. (2008). Nudge: Improving Decisions About Health, Wealth, and Happiness. Yale University Press.
- Simon, H. A. (1947). Administrative Behavior: A Study of Decision-Making Processes in Administrative Organizations. Macmillan.
- Kahneman, D. (2011). Thinking, Fast and Slow. Farrar, Straus and Giroux.
- Hofstede, G. (2001). Culture’s Consequences: Comparing Values, Behaviors, Institutions and Organizations Across Nations. Sage Publications.
- Sterman, J. D. (2000). Business Dynamics: Systems Thinking and Modeling for a Complex World. McGraw-Hill.
- Teece, D. J. (1997). Dynamic Capabilities and Strategic Management. Strategic Management Journal, 18(7), 509-533.
- O’Reilly, C. A., & Tushman, M. L. (2004). The Ambidextrous Organization. Harvard Business Review, 82(4), 74-81.
- Senge, P. M. (1990). The Fifth Discipline: The Art and Practice of the Learning Organization. Doubleday.
- Belton, V., & Stewart, T. J. (2002). Multiple Criteria Decision Analysis: An Integrated Approach. Springer.
- Clemen, R. T., & Reilly, T. (2013). Making Hard Decisions with Decision Tools. Cengage Learning.
- Rawls, J. (1971). A Theory of Justice. Harvard University Press.
- Rescher, N. (2003). Epistemology: An Introduction to the Theory of Knowledge. State University of New York Press.
- Provost, F., & Fawcett, T. (2013). Data Science for Business: What You Need to Know about Data Mining and Data-Analytic Thinking. O’Reilly Media.
- O’Neil, C. (2016). Weapons of Math Destruction: How Big Data Increases Inequality and Threatens Democracy. Crown Publishing.
- Axelrod, R. (1984). The Evolution of Cooperation. Basic Books.
- Stone, D. (2002). Policy Paradox: The Art of Political Decision Making. W.W. Norton.
- Sweller, J. (1988). Cognitive Load During Problem Solving: Effects on Learning. Cognitive Science, 12(2), 257-285.
- Tversky, A., & Kahneman, D. (1974). Judgment under Uncertainty: Heuristics and Biases. Science, 185(4157), 1124-1131.
- Chopra, S., & Meindl, P. (2016). Supply Chain Management: Strategy, Planning, and Operation. Pearson.
- Bertsimas, D., Brown, D. B., & Caramanis, C. (2011). Theory and Applications of Robust Optimization. SIAM Review, 53(3), 464-501.
- Putnam, R. D. (2000). Bowling Alone: The Collapse and Revival of American Community. Simon & Schuster.
Definitions
- Choice architecture: The design of environments in which people make decisions, structured to influence their choices (Thaler & Sunstein, 2008).
- Dynamic capabilities: The ability of an organization to adapt its resources and processes to changing environments (Teece, 1997).
- Ambidextrous organizations: Organizations that balance exploration and exploitation to achieve both innovation and efficiency (O’Reilly & Tushman, 2004).
- Multi-criteria decision analysis (MCDA): A decision-making method that evaluates and compares multiple options based on predefined criteria (Belton & Stewart, 2002).
- Decision fatigue: The decline in decision quality due to mental exhaustion from making numerous decisions (Baumeister & Tierney, 2011).
- Causal loop diagram: A visual representation of feedback loops within a system, used to analyze dynamic interactions (Senge, 1990).
- Cultural dimensions theory: A framework for understanding cultural differences across six dimensions, including individualism and power distance (Hofstede, 2001).
- Social capital: The networks and relationships that facilitate cooperation and trust within organizations (Putnam, 2000).
Decision Governance
This text is part of the series on the design of decision governance. Other texts on the same topic are linked below. This list expands as I add more texts on decision governance.
Introduction to Decision Governance
- What is Decision Governance?
- What Is a High Quality Decision?
- When is Decision Governance Needed?
- When is Decision Governance Valuable?
- How Much Decision Governance Is Enough?
- Are Easy Options the Likely Choice?
- Can Decision Governance Be a Source of Competitive Advantage?
Stakeholders of Decision Governance
- Who Is Responsible for Decision Governance in a Firm?
- Who are the Stakeholders of Decision Governance?
- What Interests Do Stakeholders Have in Decision Governance?
- What the Organizational Chart Says about Decision Governance
Foundations of Decision Governance
- How to Spot Decisions in the Wild?
- When Is It Useful to Reify Decisions?
- Decision Governance Is Interdisciplinary
- Individual Decision-Making: Common Models in Economics
- Group Decision-Making: Common Models in Economics
- Individual Decision-Making: Common Models in Psychology
- Group Decision-Making: Common Models in Organizational Theory
Role of Explanations in the Design of Decision Governance
- Explaining Decisions
- Simple & Intuitive Models of Decision Explanations
- Max(Utility) from Variety & Taste
- Expected Uncertainty to Unexpected Utility
- Perceptiveness & Experience Shape Rapid Choices
Design of Decision Governance
- The Design Space for Decision Governance
- Decision Governance Concepts: Situations, Actions, Commitments and Decisions
- Decision Governance Concepts: Outcomes to Explanations
- Slow & Complex Decision Governance and Its Consequences
Design Parameters of Decision Governance
Design parameters of decision governance, or factors that influence decision making and that we can influence through decision governance:
- Factors influencing how an individual selects and processes information
- Factors influencing information the individual can gain access to
Factors influencing how an individual selects and processes information in a decision situation, including which information the individual seeks and selects to use:
- Psychological factors, which are determined by the individual, including their reaction to other factors:
- Attention:
- Memory:
- Mood
- Emotions:
- Temporal Distance:
- Social Distance:
- Expectations
- Uncertainty
- Attitude
- Values
- Goals:
- Preferences
- Competence
- Social factors, which are determined by relationships with others:
- Impressions Of Others: How They Influence Decisions And How To Regulate Them
- Reputation
- Social Hierarchies:
- Social Hierarchies: Why They Matter for Decision Governance
- Social Hierarchies: Benefits and Limitations in Decision Processes
- Social Hierarchies: How They Form and Change
- Power: Influence on Decision Making and Its Risks
- Power: Relationship to Psychological Factors in Decision Making
- Power: Sources of Legitimacy and Implications for Decision Authority
- Power: Stability and Destabilization of Legitimacy
Factors influencing information the individual can gain access to in a decision situation, and the perception of possible actions the individual can take, and how they can perform these actions:
- Governance factors, which are rules applicable in the given decision situation:
- Incentives
- Rules
- Rules-in-use
- Rules-in-form
- Institutions
- Technological factors, or tools which influence how information is represented and accessed, among others, and how communication can be done
- Environmental factors, or the physical environment, humans and other organisms that the individual must and can interact with
Change of Decision Governance
- Public Policy and Decision Governance:
- Compliance to Policies:
- Transformation of Decision Governance
- Mechanisms for the Change of Decision Governance
