How To Measure The Quality of Decision Governance?
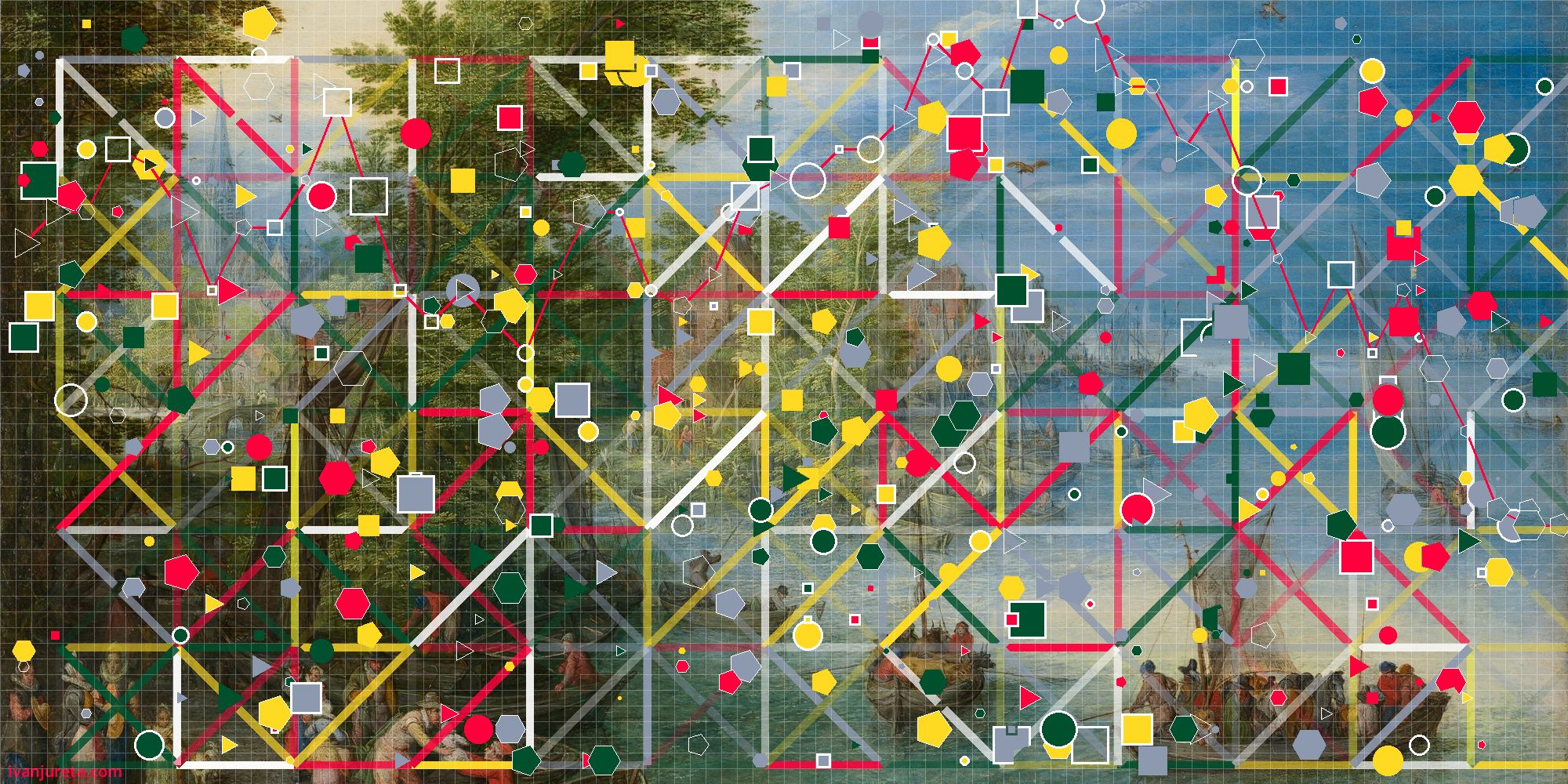
Governance has a cost, so how do we know that governance is beneficial? Public indices such as the Worldwide Governance Indicators (WGI) and the Varieties of Democracy project are interesting examples of frameworks for how to measure decision governance. This text adapts their ideas to decision governance in a firm, and then applies the resulting framework to a concrete case: a five‑stage process for allocating capital to infrastructure projects.
This text is part of the series on decision governance. Decision Governance is concerned with how to improve the quality of decisions by changing the context, process, data, and tools (including AI) used to make decisions. Understanding decision governance empowers decision makers and decision stakeholders to improve how they make decisions with others. Start with “What is Decision Governance?” and find all texts on decision governance here.
1. Start with a normative anchor
Public‑sector metrics are not value‑free. WGI begins from liberal‑institutional values such as voice and rule of law (Kaufmann et al., 2024), while the Quality‑of‑Government school centres on impartiality (Rothstein & Teorell, 2008). Values in a firm must be equally explicit. A useful anchor is the six‑point decision‑quality standard developed by Spetzler and co‑authors—clarity of purpose, creative alternatives, reliable information, sound reasoning, commitment to action and aligned values (Spetzler et al., 2016). These principles map neatly onto corporate versions of “voice”, “effectiveness” and “control of corruption”.
2. Define measurable pillars
Borrowing WGI’s multidimensional structure, a corporate Decision Governance Index (DGI) can be organised around five pillars shown below.
Pillar | Public analogue | Typical corporate indicator (examples) |
---|---|---|
Participation & transparency | Voice and accountability | % of material investment proposals that receive cross‑functional review; intranet publication rate of decision memos |
Process capability | Government effectiveness | Median cycle‑time from proposal to approval (“decision velocity”) (Li et al., 2023) |
Regulatory & ethical compliance | Rule of law; control of corruption | Share of strategic decisions reviewed for ESG impact under ISO 37005:2024 guidance (ISO, 2024a) |
Strategic alignment | Regulatory quality | Share of capital allocated to projects meeting hurdle rates derived from stated strategy |
Learning & adaptability | Political stability/instability (resilience) | % of major decisions that undergo post‑implementation review within 12 months; rate of corrective action taken |
The mix reflects two conditions absent in state governance: shareholder primacy and profit orientation. Strategic alignment therefore substitutes for the macro‑regulatory dimension, while learning & adaptability captures the premium that competitive markets place on iterative improvement.
3. Combine three data streams
Public indices rely on a blend of objective data, expert codings and perception surveys to minimise bias and fill gaps (Kaufmann et al., 2024). The same triangulation works in firms:
- Process analytics from enterprise‑resource‑planning systems record timestamps, approval hierarchies and exception flags, generating hard evidence for velocity and compliance.
- Expert audits—for instance an ISO‑aligned governance audit (ISO, 2024a)—score qualitative elements such as clarity of mandate, segregation of duties and conflict‑of‑interest controls.
- Stakeholder surveys capture perceived fairness and clarity of decisions among managers and staff; they correspond to the perception components embedded in WGI and V‑Dem.
Bayesian aggregation methods used by WGI adjust for source reliability; open‑source statistical packages now allow in‑house risk or data‑science teams to replicate those corrections, yielding confidence intervals rather than point estimates.
4. Weight and normalise
The public literature shows that rankings are highly sensitive to weights. Kaufmann et al. (2024) distribute them evenly to avoid normative claims; the Sustainable Governance Indicators adjust weights to reflect OECD priorities. Boards should follow three rules.
- Tie weights to strategy. A pharmaceutical group racing to file patents may put 30 per cent of the DGI on decision velocity; a utility under regulatory scrutiny may emphasise compliance.
- Disclose the weighting logic. Transparency curbs political gaming inside the firm—an internal echo of the “halo” problem in perception‑based country scores.
- Re‑calibrate annually. Strategy and risk appetite evolve; so should the index.
Normalisation is equally important. Firms can rescale each metric to a 0–100 scale using industry benchmarks or peer medians where data are available, then aggregate by weighted average.
5. Drill down with stage‑specific scorecards
Composite indices reveal patterns, not root causes. Governments therefore pair WGI with specialised tools such as the Public Expenditure and Financial Accountability (PEFA) framework. Firms can mirror that logic by attaching stage scorecards to the five‑stage decision process (reaction, explanation, search, decision, action).
Stage | Diagnostic metric | Analogue to public tool |
---|---|---|
Reaction | % of triggers logged in issue‑tracker; average detection‑to‑registration lag | Early‑warning and surveillance indicators in crisis governance |
Explanation | Completeness score of causal analysis (checklist audit) | Policy‑diagnostic tools in development evaluation |
Search | Number and diversity of alternatives generated per decision; use of external benchmarking | Regulatory impact‑assessment templates |
Decision | Ratio of NPV‑positive options rejected; adherence to decision‑rights matrix | Budget‑credibility tests in PEFA |
Action | On‑time, on‑budget implementation rate; post‑audit score | Implementation trackers in SDG 16 reporting |
Because each metric sits close to managerial action, stage scorecards guide targeted interventions—process redesign, training or data‑quality improvements (Serra et al., 2024)—in ways a composite DGI cannot.
6. Example: allocating capital to infrastructure projects
Large infrastructure schemes suffer from well‑documented optimism bias—average cost overruns hover around 39 per cent, while forecast benefits are routinely exaggerated (Flyvbjerg & Stewart, 2022). A disciplined measurement regime therefore pays for itself quickly. Table 1 adapts the generic stage scorecards to a board‑approved process that allocates, say, US $800 million annually across a portfolio of logistics hubs, data centres and renewable‑energy assets.
Stage | Capital‑allocation trigger | Stage‑specific indicator | Data source | Target (illustrative) |
---|---|---|---|---|
Reaction | Demand forecast, regulatory change, asset failure | % of triggers documented within five working days; monetary value of unrecorded triggers found ex post | ERP incident module; internal audit | ≥95 %; <2 % of portfolio value |
Explanation | Internal business case template | Proportion of cases with independent reference‑class forecasting; variance between reference‑class and in‑house forecasts | Cost‑benchmark database; finance review | 100 %; variance <15 % |
Search | Longlist of design‑finance‑operate options | Average number of viable options reaching stage‑gate 2; diversity index of option attributes (PPP vs. on‑balance‑sheet, modular vs. greenfield) | Stage‑gate system; procurement records | ≥4 options |
Decision | Investment committee approval | Time‑weighted portfolio NPV / capital budget (“strategic‑fit yield”); % of decisions deviating from hurdle rate without documented rationale | Capital‑planning tool; IC minutes | ≥1.10; ≤5 % |
Action | Construction and commissioning | Schedule performance index (SPI); benefit‑realisation rate 24 months post‑launch | PMO dashboard; post‑implementation reviews | SPI ≥ 0.90; benefits ≥80 % of plan |
Two points deserve emphasis.
- First, measure ex‑ante and ex‑post. Reference‑class forecasting at the Explanation stage tackles bias before approval (Lovallo & Kahneman, 2020), while benefit‑realisation audits at Action feed data back into the reference class.
- Second, link indicators to incentives. Project sponsors earn variable pay not on capital committed but on strategic‑fit yield and post‑launch benefit capture, curbing gold‑plating and “build‑fast” politics.
7. Governance of the index itself
“Gaming the metric” is as old as metrics. Public authorities mitigate manipulation by outsourcing data collection and publishing methods. Inside companies the equivalent safeguards are:
- Ownership by an assurance function (internal audit or risk) rather than by operational managers.
- Annual external validation by a professional‑services firm or an academic partner.
- Board‑level dashboard reporting the DGI and stage scorecards side‑by‑side with financial KPIs, cementing their relevance.
ISO 37005:2024 explicitly recommends that governing bodies oversee indicator design and review their continued fitness for purpose (ISO, 2024a), echoing the principle of meta‑governance in public administration.
8. Limits and future refinements
Just as WGI blends perceptions and facts, the DGI must accept a degree of subjectivity. Decisions about strategic alignment or the sufficiency of alternatives are partly judgement calls. Precision improves over time as firms accumulate post‑decision reviews—the private equivalent of “learning loops” in adaptive governance.
Second, cross‑firm comparability remains awkward because business models differ. A practical compromise is to benchmark within industry cohorts using publicly available governance disclosures (board diversity, ESG integration) and voluntary standards such as ISO’s IWA 48 for ESG practice (ISO, 2024b).
Finally, new research on intuitive strategic decision‑making reminds us that speed and comprehensiveness trade off in complex settings (Top Management Intuition Research Group, 2024). The index should therefore track both and allow for context‑specific optimisation rather than prescribing a single “best” level.
9. Conclusion
Public‑sector experience shows that a robust governance metric must (i) rest on a clear normative anchor, (ii) cover multiple pillars, (iii) blend data sources and (iv) remain open to scrutiny. By adapting these principles—anchoring on decision‑quality theory, defining a five‑pillar DGI, triangulating process analytics with expert audits and surveys, and guarding against metric manipulation—firms can routinise disciplined, transparent and adaptive decision governance. The worked example demonstrates how the same logic yields hard‑edged, stage‑specific metrics for capital allocation. In time the index should become as indispensable to senior management as the balance sheet: a concise statement of whether the organisation is likely to keep making good decisions before it discovers that it has not.
Definitions
- Decision governance: The structures, processes and behavioural norms that determine how decisions are proposed, evaluated, authorised and reviewed within an organisation. (ISO, 2024a)
- Decision quality: The degree to which a decision meets six conditions: clear frame, creative alternatives, meaningful information, sound reasoning, commitment to action and aligned values. (Spetzler et al., 2016)
- Composite index: A single score that aggregates normalised indicators, often with explicit weights, to summarise a multidimensional concept. (Kaufmann et al., 2024)
- Decision velocity: The elapsed time between initial proposal registration and formal approval, adjusted for decision significance. (Li et al., 2023)
- Meta‑governance: The governance of governance: oversight arrangements that ensure governance frameworks remain effective and legitimate over time. (ISO, 2024a)
References
- Bertelsmann Stiftung. 2024. Sustainable Governance Indicators: Methodology 2024. Gütersloh: Bertelsmann Stiftung.
- Flyvbjerg, B., & Sovacool, B. K. 2023. “Megaprojects and risk: a research agenda for infrastructure investors.” Energy Policy 165: 112 959.
- Fukuyama, F. 2013. “What is governance?” Governance 26 (3): 347‑368.
- IBM Institute for Business Value. 2023. The Enterprise Guide to AI Governance. Armonk, NY.
- ISO. 2024a. ISO 37005:2024 Governance of Organisations — Indicators for Effective Governance. Geneva: International Organization for Standardization.
- ISO. 2024b. IWA 48:2024 Environment, Social and Governance (ESG) — Principles and Practice. Geneva: International Organization for Standardization.
- Kaufmann, D., Kraay, A., & Mastruzzi, M. 2024. The Worldwide Governance Indicators: Methodology and 2024 Update. World Bank Policy Research Working Paper 10952. Washington, DC: World Bank.
- Li, S., Chen, J., & Huang, X. 2023. “The mediating roles of decision speed and comprehensiveness.” Journal of Organizational Computing and Electronic Commerce 33 (2): 142‑165.
- Mo Ibrahim Foundation. 2024. Ibrahim Index of African Governance 2024 Report. London: Mo Ibrahim Foundation.
- OECD. 2023. Government at a Glance 2023. Paris: OECD Publishing.
- PEFA Secretariat. 2016. PEFA Framework for Assessing Public Financial Management. Washington, DC: World Bank.
- Project Management Institute (PMI). 2021. Practice Standard for Benefits Realization Management. Newtown Square, PA: PMI.
- Rothstein, B., & Teorell, J. 2008. “What is quality of government? A theory of impartial government institutions.” Governance 21 (2): 165‑190.
- Serra, F., et al. 2024. “Can big data improve firm decision quality? The role of data quality and diagnosticity.” Information & Management (forthcoming).
- Spetzler, C., Winter, H., & Meyer, J. 2016. Decision Quality: Value Creation from Better Business Decisions. Hoboken, NJ: Wiley.
- Top Management Intuition Research Group. 2024. “Exploring intuition in strategic decision‑making.” Industrial Marketing Management 119: 34‑46.
- United Nations Development Programme (UNDP). 2022. Evaluating Progress Towards SDG 16: Effective Governance and Peaceful Societies. New York: UNDP.
- Varieties of Democracy Institute. 2025. V‑Dem Dataset v15 and Democracy Report 2025. Gothenburg: University of Gothenburg.
Decision Governance
This text is part of the series on the design of decision governance. Other texts on the same topic are linked below. This list expands as I add more texts on decision governance.
- Introduction to Decision Governance
- Stakeholders of Decision Governance
- Foundations of Decision Governance
- How to Spot Decisions in the Wild?
- When Is It Useful to Reify Decisions?
- Decision Governance Is Interdisciplinary
- Individual Decision-Making: Common Models in Economics
- Group Decision-Making: Common Models in Economics
- Individual Decision-Making: Common Models in Psychology
- Group Decision-Making: Common Models in Organizational Theory
- Role of Explanations in the Design of Decision Governance
- Design of Decision Governance
- Design Parameters of Decision Governance
- Factors influencing how an individual selects and processes information in a decision situation, including which information the individual seeks and selects to use:
- Psychological factors, which are determined by the individual, including their reaction to other factors:
- Attention:
- Memory:
- Mood:
- Emotions:
- Commitment:
- Temporal Distance:
- Social Distance:
- Expectations
- Uncertainty
- Attitude:
- Values:
- Goals:
- Preferences:
- Competence
- Social factors, which are determined by relationships with others:
- Impressions of Others:
- Reputation:
- Promises:
- Social Hierarchies:
- Social Hierarchies: Why They Matter for Decision Governance
- Social Hierarchies: Benefits and Limitations in Decision Processes
- Social Hierarchies: How They Form and Change
- Power: Influence on Decision Making and Its Risks
- Power: Relationship to Psychological Factors in Decision Making
- Power: Sources of Legitimacy and Implications for Decision Authority
- Power: Stability and Destabilization of Legitimacy
- Power: What If High Decision Authority Is Combined With Low Power
- Power: How Can Low Power Decision Makers Be Credible?
- Social Learning:
- Psychological factors, which are determined by the individual, including their reaction to other factors:
- Factors influencing information the individual can gain access to in a decision situation, and the perception of possible actions the individual can take, and how they can perform these actions:
- Governance factors, which are rules applicable in the given decision situation:
- Incentives:
- Incentives: Components of Incentive Mechanisms
- Incentives: Example of a Common Incentive Mechanism
- Incentives: Building Out An Incentive Mechanism From Scratch
- Incentives: Negative Consequences of Incentive Mechanisms
- Crowding-Out Effect: The Wrong Incentives Erode the Right Motives
- Crowding-In Effect: The Right Incentives Amplify the Right Motives
- Rules
- Rules-in-use
- Rules-in-form
- Institutions
- Incentives:
- Technological factors, or tools which influence how information is represented and accessed, among others, and how communication can be done
- Environmental factors, or the physical environment, humans and other organisms that the individual must and can interact with
- Governance factors, which are rules applicable in the given decision situation:
- Factors influencing how an individual selects and processes information in a decision situation, including which information the individual seeks and selects to use:
- Change of Decision Governance
- Public Policy and Decision Governance:
- Compliance to Policies:
- Transformation of Decision Governance
- Mechanisms for the Change of Decision Governance