What Does a Training Data Market Mean for Authors?
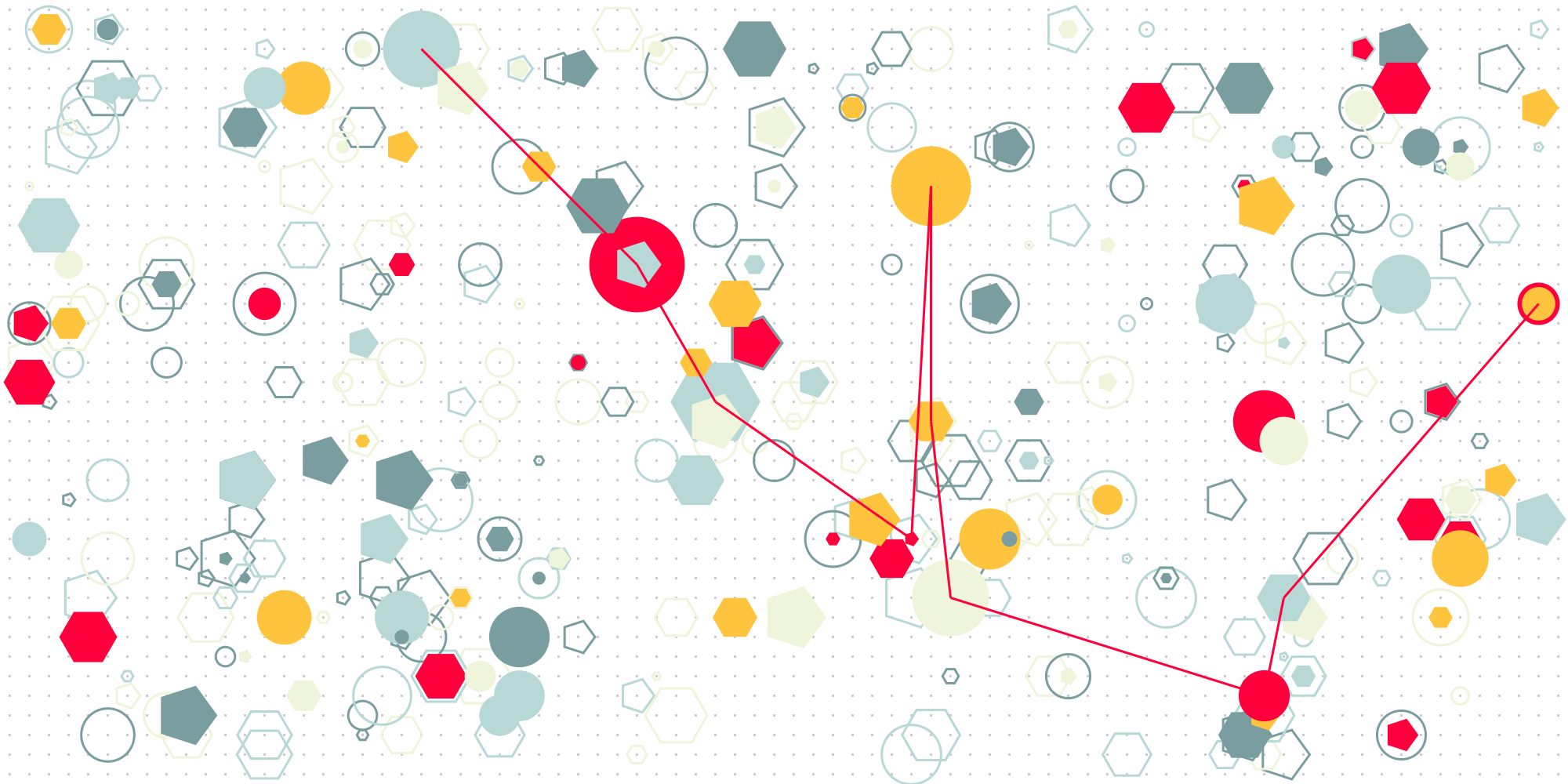
If any text can be training data for a Large Language Model, then any text is a training dataset that can be valued through a market for training data.
Which datasets have high value? Wikipedia, StackOverflow, Reddit, Quora are examples that have value for different reasons, that is, because they can be used to train AI that serves different purposes. For them to have high value, they need to be split from large-scale crawled web data – and consequently, it should not be possible to freely crawl them.
Note that Wikipedia can be crawled, while StackOverflow publishes its data every few months. It is not clear why this makes economic sense, other than to support research – when the research starts yielding products that themselves generate value, e.g., OpenAI’s products, then the incentive to allow crawling, or the self-publishing of data is likely to be less interesting, unless property rights can be exercised (see the note here).
A category of datasets that will have very high value are text data held by academic publishers (Elsevier, Springer, etc.). Think about how that data is assembled: peer-reviewed research papers are data in the context of this note, and such content involves significant public and/or private investment to be generated, then additional investment of time from select experts to be reviewed and accepted for publication.
In all cases above, Wikipedia, StackOverflow, Elsevier, and such, authors of content that these assemble and publish are individuals – they are part of various expert communities, including in engineering and science.
A question that will need resolution over the coming years, as revenues from LLM use continue to increase, is how to incentivize these individuals to continue creating content, and contributing to increase its quality (e.g., through peer-review)?
Addressing this question will require some form of compensation for contributors. It may be reputational, as it is now; that would require different AI products than today – products which could, for example, attribute their output to named authors. It may be financial, in which case authors need to receive compensation, e.g., royalties, whenever their content was used to compute an answer valued by AI product’s users.
An important implication of the above is that a functioning market for training datasets requires a very different Internet than today – one where property rights of content creators are supported by appropriate and better enforced content licensing rules, different Terms and Conditions on platforms/services publishing content, and much better controls for authors over the use of their content. This also begs the question of how such rules will handle content created in the past.
All content creators have a clear incentive to be interested in how this will develop, including those funding them – in the case of research content, it is not only the scientist who needs to have a say, it is also the funding agency, which often uses public funds.