Which Problems Is It Hard to Design AI for?
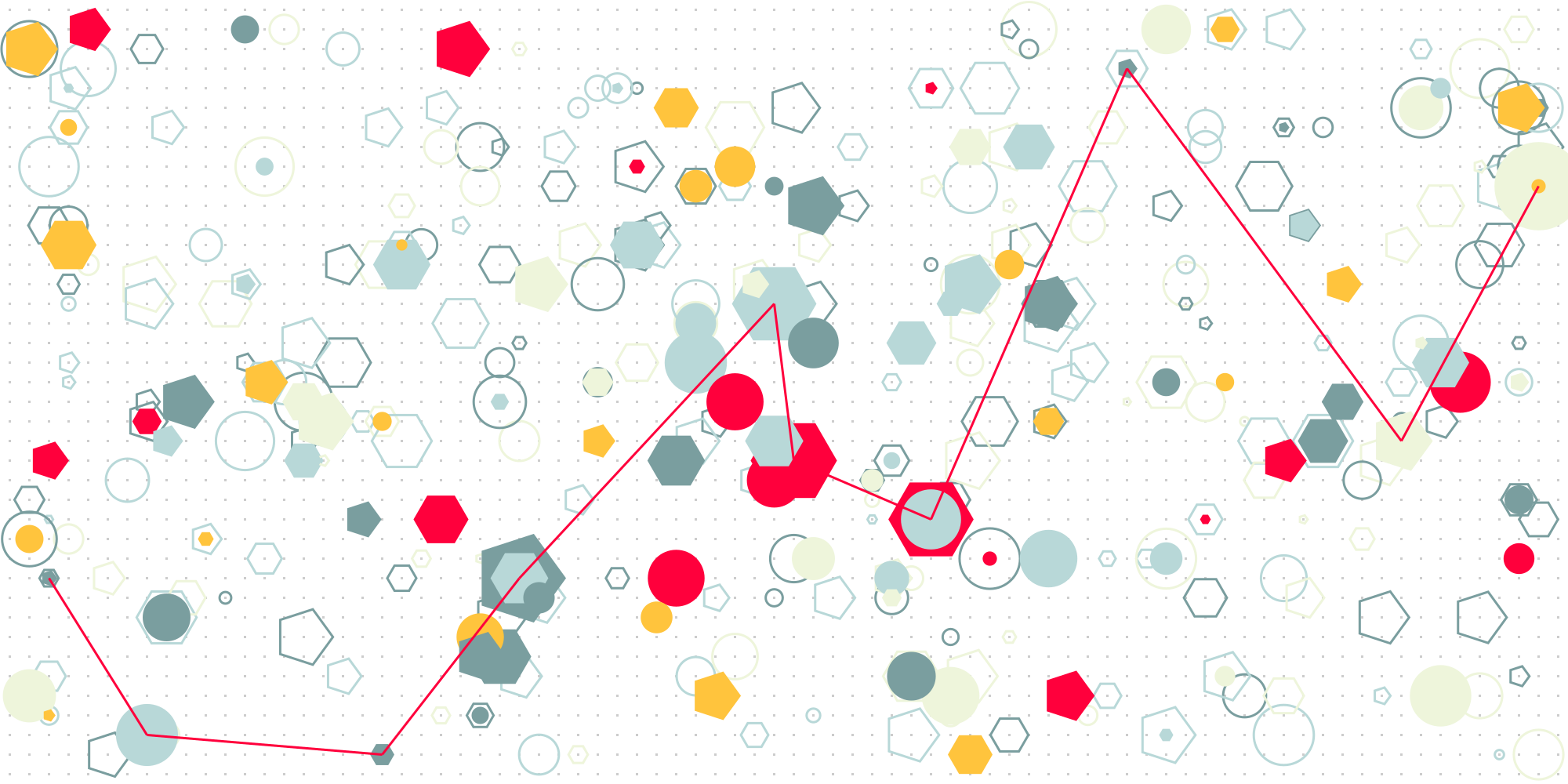
The less data there is, or the lower quality the data that is available, the more difficult it is to build AI based on statistical learning. For scarce data domains, the only way to design AI is to elicit knowledge from experts, design rules that represent that knowledge, parameterize them so that they apply to more cases.
AI based on expert rules is relatively more expensive to design than AI based on statistical learning, the more data there is to train the AI, and the higher the quality of that data. An extreme example would be if someone wanted to make an AI system comparable to any version of ChatGPT, and they had no access to crawled large scale Internet data, but instead had to elicit information from people. This is impossible, as it would imply eliciting all sorts of information that accumulated online over the last few decades. Put another way, many of the currently interesting AI systems available to consumers, and those based on Large Language Models in particular, depend heavily on Internet content, and on it being low cost to use for training.
There’s an important implication of this reliance on Internet content, and its scale in particular: most knowledge or problem domains for which content to derive patterns from is scarce, will lead to AI systems that cannot perform at comparable levels of sophistication as those trained on general purpose Internet content.
A few hypotheses, then, about the enterprise AI market, or the market for AI systems trained on enterprise data:
- Adoption of enterprise AI will be lower than the adoption of general purpose AI: The percentage of staff in an organization who use that organization’s enterprise AI is likely going to be lower than the percentage of people using general purpose AI systems built on Internet data.
- Lower adoption of enterprise AI will lead to the lower impact of AI on headcount, in particular for jobs that involve making impactful decisions.
- General purpose AI, such as ChatGPT and similar, will have more impact on headcount than enterprise AI trained on enterprise data and content, and the mechanism for that impact will be the application of general purpose AI to repetitive information management tasks, in particular tasks involving search and synthesis of information that is not specific to the given organization.
I look forward to being proven wrong about these, as that is the more interesting outcome.