How to Make GenAI Better Faster? Authorship + Community + Credibility
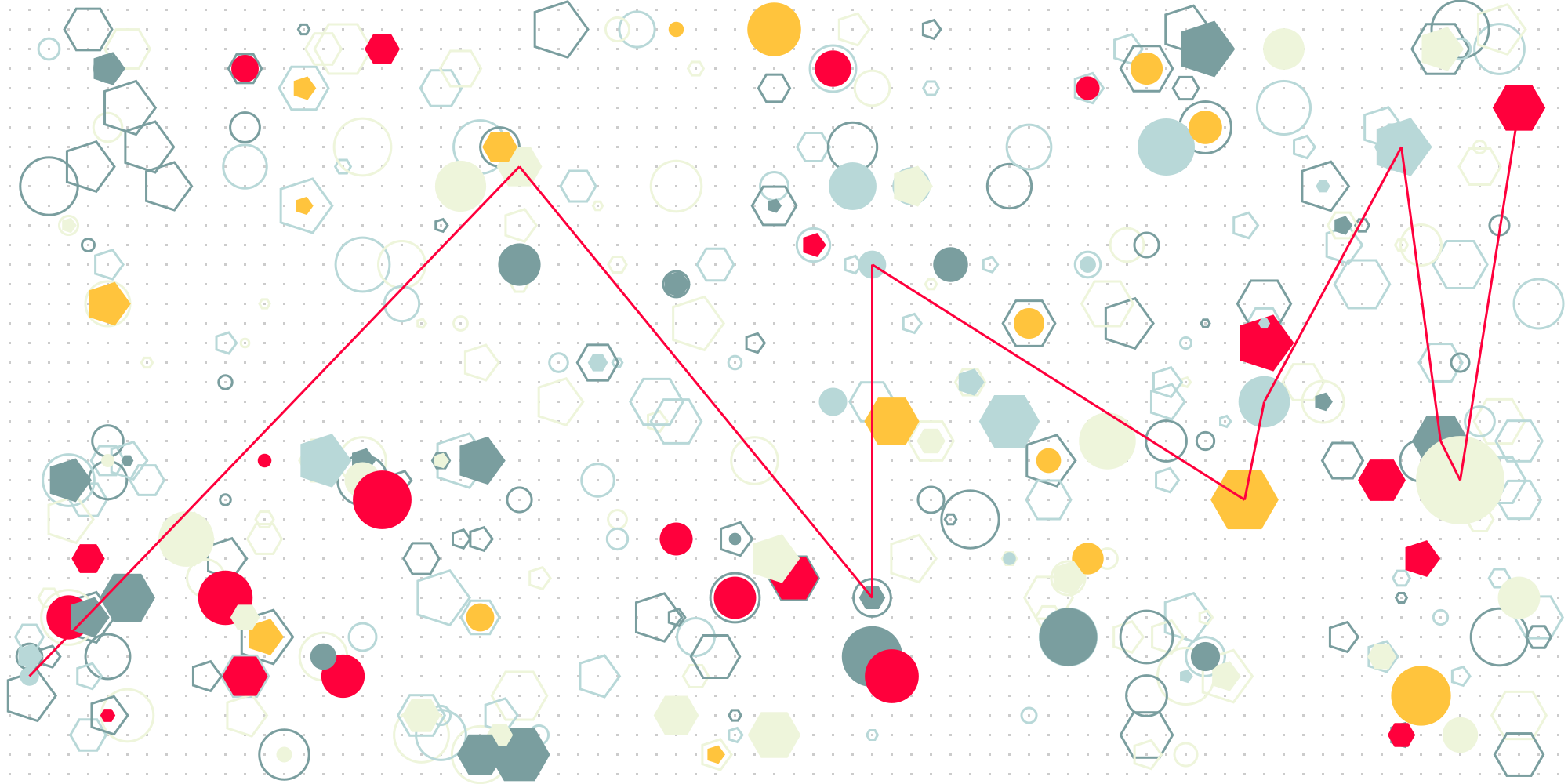
We should reduce the cost of authorship and create an incentive mechanism that generates and assigns credibility to authors in a community. In simpler terms, it should be much easier than today to contribute to the improvement of GenAI such as ChatGPT, and when doing so, the contribution needs to be visible to and value thereof recognizable by a community of peer authors.
The idea came from thinking about Benkler’s Wealth of Networks, and fundamental differences between collaboration and contribution to content on the pre-GenAI Internet (think Wikipedia, open source software) and the current Internet that is only starting to experience and be changed by GenAI. I wrote about AI governance (articles are here), but most of it is rather narrow in its purpose, aiming to increase safety and accuracy of AI-generated content. That’s valuable, but limited, because it relies on rules that mostly inhibit, or at least slow down change. I’m interested in this problem of better faster GenAI, because it is a governance problem.
Take Wikipedia. People write, others review their contributions, and some contributions become part of the article on the given topic. Whether a contribution is added depends on the review by those who previously acquired credibility in the community of authors. Anyone can become an author, and their ability to match their contributions to the expectations of those reviewing them will determine the credibility these new authors acquire, and how fast they acquire it. The more authors, the more active they are, the more valuable Wikipedia is to authors and readers. Network effects, themselves a result of a great incentive mechanism.
It is not obvious at all how that mechanism could be reproduced for GenAI, and what effect it would have. Before we go there, we need to review fundamental differences between sources of information like Wikipedia, and a GenAI, say, ChatGPT. Despite differences in the user experience of the two, they both share a critical function for their users, which is that they go to these services to get answers to questions. (I’m not saying this is the only purpose for both, but this is the use case I’m focusing on.)
Consider what you need to know, in order to contribute to Wikipedia. One, you need to know something about the topic you want to contribute to. Two, editing an article is rather straightforward, the user interface makes this easy, and there are straightforward instructions about what happens after edits are made. The outcome is easy to see after a decision on edits has been made.
Contrast this with what you need to know to contribute to improving answers a GenAI product provides. There are multiple ways of doing this, and the important point is that all these ways to make improvements to GenAI currently require specialized knowledge that takes years to acquire in addition to the knowledge of the topic you want to improve answers (AI’s responses) about. Here are ways to improve GenAI, and the issue I just highlighted is obvious in each case.
- You can improve training datasets, which for common GenAI products means that you need to publish content on the Internet which they use as training data. Contributing to Wikipedia is an example. One problem with this is that while your contribution may lead you to gain credibility on Wikipedia, this is lost in the GenAI product, because it will likely not cite authors at that level of detail. The issue is slightly less problematic for researchers publishing in academic publications, as peer reviewed publications are referenced by some GenAI products if you request that through their prompt.
- You can do the most difficult thing, which is to do research on new algorithms which are used to train models. In this case, the community that would recognize your work is going to be that which is developing the product. This is less of an issue in the case of open source AI, and again, if you do academic research.
- You can customize existing algorithms, which is approximately the same as above.
- You can specialize existing models, by adding rules that, say, filter responses, and, or you can curate differently the training data, such as select only some of it to train the AI.
Today, any approach where you are contributing to training data comes with the problem that it is difficult to highlight your contribution through the responses GenAI provides. This is not surprising because the relationship between inputs and outputs in a GenAI system is not trivial enough that it is feasible to recognize all inputs that contributed in a response to a question. Think about the billions of parameters of current models.
Any approach that involves you developing new algorithms and models, or customizing these, comes with the issue that the learning curve is long and steep. It makes it highly unlikely that many people can do it, or to put it differently, reduces the number of people who could contribute.
To simplify considerably, the situation today is such that to contribute to making GenAI systems better, you either need to work in the firm or organization that makes and commercializes or publishes it, and, or you need to contribute to sources used as training data.
How could we then achieve a similar effect that Wikipedia is an example of, but now for GenAI? How can we design a mechanism that would enable many more people to contribute, easily and cheaply, without much learning, to improve GenAI responses?
To do that, we need the following.
- There needs to be the role of author. Authors need to be able to contribute so that their contribution can be tracked and that it is visible – they need to be credited as authors.
- If an author’s contribution is valuable to others, they need to be able to show recognition for it, there needs to be a way to measure credibility of authors and attribute credibility based on contributions.
- If an author’s contribution is not valuable, or is destructive, that should affect their credibility.
- There need to be ways for the author community to communicate and exchange advice and experience about their contributions, so that they can learn from each other.
- The system that authors are contributing to needs to be such that authors trust that it will last long enough for them to want to invest their effort to contribute. (This could be solved by having multiple systems be able to use the same contributions, similarly to how they use similar training data.)
This gets us back to the types of authors that already exist, and who are contributing in ways I mentioned earlier. Having a solution for the five requirements above would mean increasing the number of authors considerably, and with that, accelerate improvements of GenAI systems. The next problem, then, is: how?
Further Reading
- Forte, Andrea, and Amy Bruckman. “Why do people write for Wikipedia? Incentives to contribute to open–content publishing.” Proc. of GROUP 5 (2005): 6-9.
- Rafaeli, Sheizaf, and Yaron Ariel. “Online motivational factors: Incentives for participation and contribution in Wikipedia.” Psychological aspects of cyberspace: Theory, research, applications 2.08 (2008): 243-267.