Preconditions for a Market for High Quality AI Training Data
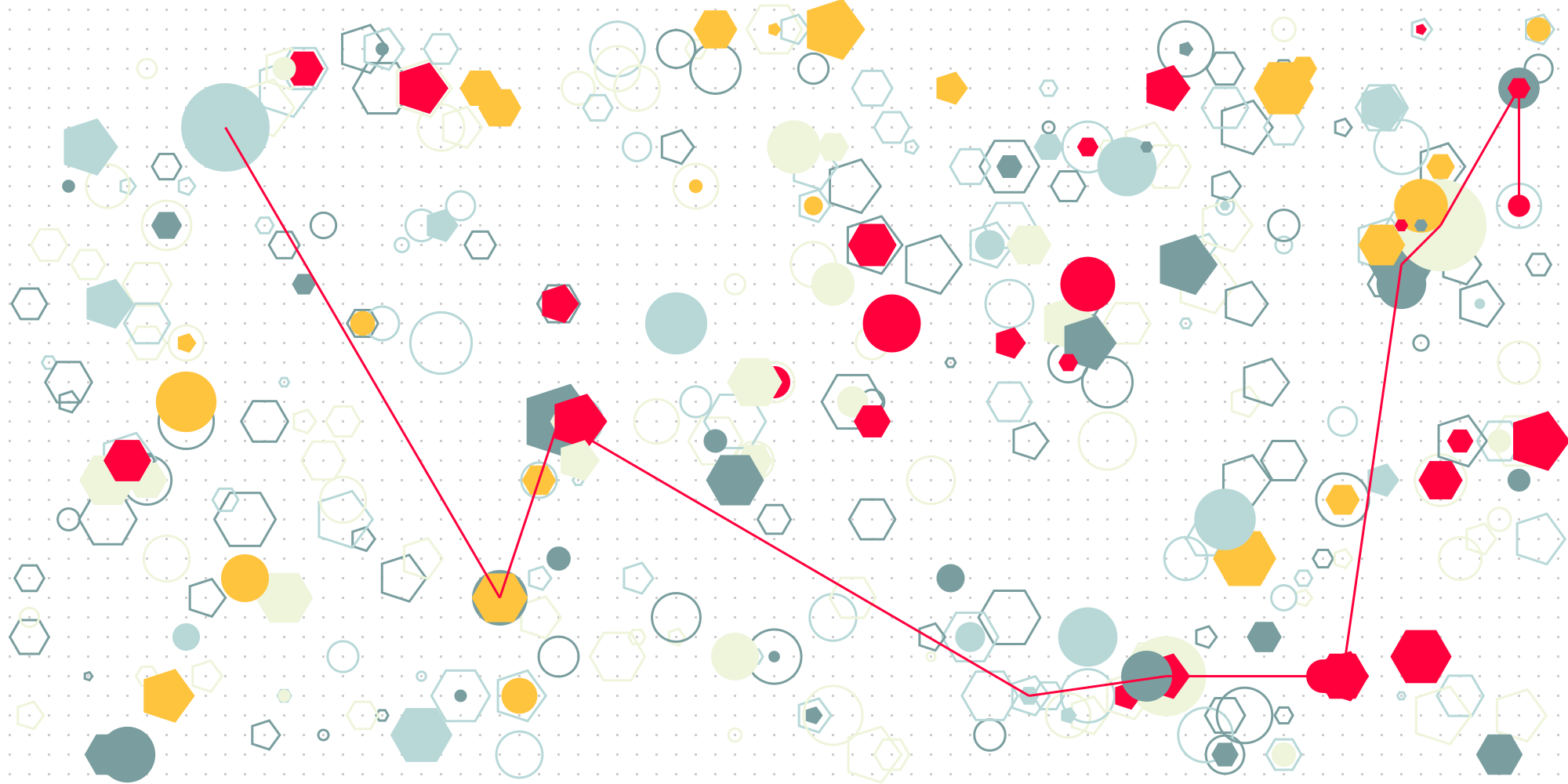
There is no high quality AI without high quality training data. A large language model (LLM) AI system, for example, may seem to deliver accurate and relevant information, but verifying that may be very hard – hence the effort into explainable AI, among others.
If I wanted accurate and relevant legal advice, how much risk would I be taking if I were to ask an LLM for it? Training data used to develop it may not be well governed: it may be hard to determine authorship and identify supporting content for a statement that the AI produced as output, to determine what alternative outputs it discarded during computation, or to know anything about the relative quality of the training data used versus some other potentially relevant training data.
AI regulations (see my notes here, and here) all recognize the importance of data governance.
For high quality training data to exist, and for it to comply with applicable AI regulations, it is necessary that there are incentives to develop such datasets (I will use “dataset” to cover both static datasets and data streams below).
Economic incentives will exist if there is a market for high quality datasets. The other extreme is that only the companies designing AI are developing proprietary datasets, which they do not make available to others.
What preconditions need to be met, for such a market for data to exist?
These preconditions are the same as those for markets for other kinds of goods (see the entry for Markets in the Stanford Encyclopedia of Philosophy, here):
- Property rights need to exist and be enforceable;
- Anti-trust regulations need to exist, to reduce the probability of concentration of power, namely monopolies and oligopolies.
The author of information represented in the dataset needs to be able to be recognized as such, and it needs to be clear what authorship means – what rights and obligations the author has over their contribution to the dataset. It needs to be clear what it means to acquire the dataset.
For example, let’s say that the author provided information that turned out to be inaccurate, and that this was established through the audit of a recommendation made by the AI that was trained on the data. This example begs the question of how to protect the author from damages due to unintended consequences – some form of professional insurance is a possible means.
There need to be institutions that enforce property rights. If the author has rights to payment for use of a dataset, then there need to exist procedures that the author can undertake, to confirm what payment they are entitled to, and to confirm and enforce their right.
Property rights beg the question of how authors can be supported in publishing datasets, which is likely to lead to specialized publishers of datasets, who would handle the technical aspects of developing and publishing a dataset, collecting payments on behalf of the author, and handle the marketing, sales, and many other work across the lifecycle of content.
For the market for high quality datasets to grow, many more conditions need to be met:
- Standardized measures of data quality are needed, as it is otherwise not possible to compare the quality of datasets, even those designed for the same purpose.
- One or multiple, and if multiple, then bridged (connected) ontologies are needed to describe the scope of a dataset, applicability, and perhaps most importantly, relationship to other, substitute and complementary datasets.
- Incentives of AI system owners and dataset authors need to be aligned, so that authors benefit more if their datasets contribute to the success of an AI trained on them. For example, if the dataset is used in an AI system, and the system is highly successful because of that dataset, the author would need to benefit more than if the same system was not as successful.
- Standards are needed for how to embed (see the note here) data governance with a dataset.
- There need to exist marketplaces that enable transactions between supply and demand of training datasets, or in other words, there needs to exist a means for demand to see supply and vice versa, and for transactions to occur.